Introduction
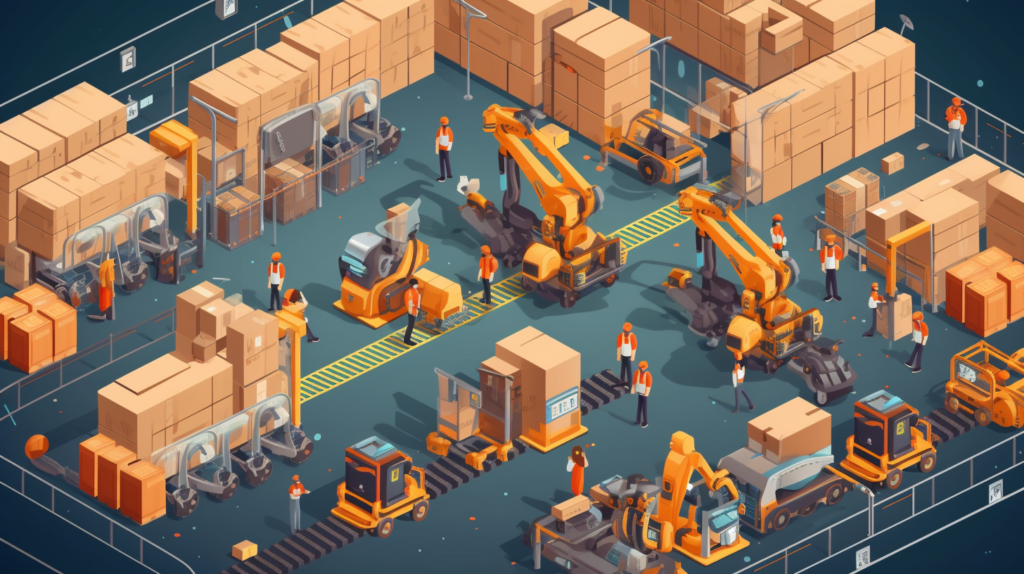
Supply chain management has a significant transformation with the rise of Artificial Intelligence (AI), delivering unprecedented efficiency and optimization. As we navigate an increasingly complex global market, businesses must adapt to stay ahead. This blog post explores AI’s role in reshaping supply chain operations, from automation and predictive analytics to sustainability, showcasing how integrating AI into your supply chain can unlock new performance levels.
Table of Contents
Key Takeaways
- AI plays a critical role in supply chain management by automating processes, predicting and forecasting demand, improving supplier relationships, and promoting sustainability.
- AI-powered tools such as predictive analytics and automation enable businesses to reduce costs, improve sustainability efforts, and enhance supplier relationships.
- Integrating AI into various aspects of the supply chain process will make businesses more efficient while contributing positively towards a greener planet.
- With continued innovation around digital transformation initiatives that leverage IoT devices for real-time tracking mechanisms that ensure on-time delivery while minimizing operational costs, the future of AI in supply chains is promising.
Role Of AI In Supply Chain Management
AI plays a critical role in supply chain management by automating processes, predicting and forecasting demand, improving supplier relationships, and promoting sustainability.
Supply Chain Automation
Supply chain automation revolutionizes how businesses manage operations by leveraging AI-powered tools and technologies. Through the integration of AI, repetitive and error-prone tasks can be performed automatically, leading to increased efficiency, productivity, and cost savings.
One notable success story comes from global shipping giant UPS; they implemented an AI-driven tool called ORION that optimizes delivery routes in real time. As a result, UPS has saved millions of miles annually on deliveries while reducing fuel consumption and carbon emissions.
Predictive Analytics And Forecasting
Predictive analytics and forecasting play a crucial role in supply chain optimization, enabling businesses to make more informed decisions based on data-driven insights.
By leveraging AI algorithms such as Seq-Seq and Auto-Encoders, companies can analyze historical demand patterns, trends, and other important factors.
For example, Gartner has reported that using machine learning for demand forecasting leads to 10% – 20% improvements in forecast accuracy. Not only does this lead to better financial performance, but it also reduces the bullwhip effect – minimizing fluctuations in inventory levels due to incorrect predictions or supplier delays.
Enhanced Supplier Relationship Management
Enhanced supplier relationship management (SRM) is vital in optimizing supply chain operations. With the integration of AI, businesses can achieve more consistent and efficient SRM by leveraging data analytics to make informed decisions based on factors such as pricing, purchase history, and sustainability.
For instance, AI-enabled software can analyze supplier performance data and rank suppliers accordingly.
In addition to improving the selection process, AI also aids in automating routine communication tasks with suppliers through Robotic Process Automation (RPA). By automating invoice sharing and payment reminders, companies can streamline vendor interactions while minimizing errors.
Furthermore, AI-enhanced tools can help monitor real-time supplier performance metrics and alert stakeholders when issues arise – ensuring timely corrective actions that contribute towards an improved overall supply chain.
Improved Sustainability
Ai plays a vital role in improving the sustainability of supply chains. AI-powered tools can help determine optimal inventory levels and region-specific demand to help business leaders make better decisions for sustainability.AI algorithms can be used to forecast demand and optimize inventory levels to reduce waste.
Moreover, reliable and automated data visual analytics platforms empowered by AI can minimize supply chain disruption and maximize business outcomes, leading to more sustainable practices.
The cost of implementing AI in the supply chain has reduced to 12% in the past two years, which benefits businesses looking to integrate sustainable practices into their operations without increasing their expenditure significantly.
Top AI Use Cases For Supply Chain Optimization
AI can automate various supply chain management processes, including back-office tasks, logistics and warehouse operations, quality checks, inventory management, and more.
Back-office Automation
Back-office automation is a crucial aspect of AI in supply chain management. Businesses can automate back-office tasks such as invoicing and documentation processing.
Automation reduces paperwork errors, increases accuracy and speed, and streamlines operations. AI tools can also assist demand forecasting by analyzing historical data and identifying patterns to predict future customer needs.
This allows businesses to allocate resources effectively, minimize waste, reduce costs, and optimize inventory levels. Additionally, using AI-driven solutions for procurement results in efficient supplier selection processes that maximize production capabilities while minimizing risk factors.
Logistics Automation
Logistics automation is one of the top AI use cases for supply chain optimization. It involves incorporating AI-powered tools to streamline the movement and delivery of goods throughout the entire supply chain network.
With logistics automation, companies can benefit from improved order accuracy, enhanced shipping speed, and reduced labor costs.
For example, companies like Amazon have already integrated robotics into their warehouses to automate inventory storage and retrieval tasks. Additionally, autonomous drones are being explored to deliver packages directly to customer doorsteps in record time while reducing human error in transportation.
Warehouse Automation
Warehouse automation is a crucial aspect of supply chain optimization, and AI plays a vital role in this area. With the help of AI-powered tools such as digital workers, cobots, warehouse robots, and autonomous vehicles, companies can significantly improve efficiency and productivity while ensuring worker safety.
For instance, computer vision systems enabled by AI can automate product quality checks to ensure that only high-quality items are shipped to customers.
In conclusion, warehouse automation has become increasingly important for businesses seeking to optimize their supply chain processes.
Automated Quality Checks
With AI, supply chain managers can automate quality checks for products in the supply chain. Using computer vision systems, AI can visually inspect and detect defects or damages in real time.
For instance, a manufacturer could use an image recognition system to check if the product is high quality before being shipped.
Moreover, when paired with IoT devices, such as sensors or cameras placed along the production line or warehouse floor, quality data can be collected 24/7 without interruptions.
Analyzing this data helps identify patterns and anomalies that provide valuable insights into improving manufacturing processes and help prevent future defects from occurring.
Automated inspections ensure consistency throughout the production process while reducing costs associated with manual checks.
Automated Inventory Management
AI-powered inventory management can provide substantial benefits for supply chains. One of the most significant advantages is the ability to analyze historical data and make demand predictions to determine optimal stock levels accurately.
This technology can help companies make better decisions about when to reorder products, minimizing the risk of overstocking or running out of critical items. It can also consider variables like delivery and lead times that may impact inventory availability.
Inventory Optimization
Effective inventory management is crucial in supply chain optimization, and AI can help achieve this through inventory optimization. With predictive analytics and machine learning algorithms, businesses can predict future demand accurately and identify slow-moving or obsolete items before they become a problem.
One excellent example of an AI-powered solution for inventory optimization is SKU (Stock Keeping Unit) rationalization. It helps eliminate unnecessary product variations, increase productivity, reduce the risk of stockouts, and minimize carrying costs while meeting customers’ needs effectively.
With these tools at their disposal, companies have much greater control over supplies and order fulfillment processes, benefiting customers and suppliers alike.
Region-specific Forecasts
AI-powered supply chain management can provide detailed region-specific demand information to help businesses make better decisions. By analyzing data collected from different regions, AI algorithms can create insights into local market trends, customer behavior, and other factors influencing demand.
For example, a food delivery service may use AI tools to analyze historical data and predict higher demand for certain types of cuisine during weekends in one region compared to others.
This insight enables them to plan ahead by stocking up on relevant ingredients or hiring extra drivers for the weekend shift.
Bullwhip Effect Prevention
The bullwhip effect causes inventory levels to fluctuate, increasing companies’ holding or storage costs. Fortunately, AI-powered forecasting tools can help reduce demand and supply fluctuations by leveraging data collected from customers, suppliers, manufacturers, and distributors to control bullwhip.
High-level accuracy in demand forecasting can help companies avoid over-production and storage costs while ensuring they have enough products to meet customer demands.
Using real-time data and advanced analytics, businesses can make informed decisions about when to place orders with suppliers or adjust production levels based on changing market conditions.
Improved Supplier Selection
AI can help improve supplier selection by enabling consistent and efficient supplier relationship management in the supply chain industry. Leveraging big data and machine learning, AI algorithms can analyze supplier data to identify patterns and provide insights that aid decision-making.
For instance, AI-based systems can monitor suppliers’ performance metrics such as delivery times, quality of goods supplied, and pricing policies.
One prominent example is Procter & Gamble (P&G), a global consumer products company that leveraged AI to transform its supplier selection process. By aggregating large volumes of procurement data from across its operations worldwide and analyzing it using advanced analytics, P&G was able to gain rapid visibility into its top-performing suppliers while identifying risk factors associated with underperformers.
This allowed the Company’s procurement team to negotiate better deals with top performers while taking action on high-risk vendors through improved communication or outright replacement.
Improved Supplier Communications
AI-powered tools can automate and streamline supplier communications, making it easier for businesses to manage their suppliers effectively. Automated communication tools help to prevent negative knock-on effects on shipment and production caused by failing to pay vendors on time.
These tools can also send timely alerts and notifications when issues arise, improving transparency in the supply chain. Additionally, AI technology can analyze supplier feedback and rank them based on performance data or other factors such as sustainability efforts or pricing.
In summary, improved supplier communications significantly benefit implementing AI in supply chain management.
Greener Transport Logistics
The transportation of goods within the supply chain is responsible for a considerable portion of global greenhouse gas emissions. However, AI-powered tools can optimize transportation routes to reduce miles traveled, resulting in greener transport logistics.
For example, DHL uses AI to optimize vehicle routes and reduce fuel consumption, lowering emissions and improving sustainability. Moreover, smart energy solutions can also help reduce carbon emissions associated with warehouse energy consumption in the supply chain.
Greener Warehousing
Implementing AI in warehousing can significantly improve sustainability, reducing the carbon footprint and energy consumption associated with warehouse operations.
For example, AI-powered inventory management systems can optimize stock levels and reduce waste by automating demand forecasting based on historical data and real-time sales information.
Some companies have successfully implemented these green solutions powered by AI technology. One such Company is DHL which uses an AI-based optimization platform for its vehicle routing software that optimizes routes to improve fuel efficiency while minimizing emissions.
This has helped the Company meet its climate protection target of reducing all logistics-related emissions to zero by 2050 while cutting transportation costs and improving service quality.
Challenges And Solutions In Implementing AI In Supply Chains
Companies face challenges when integrating AI into their supply chains, such as ensuring data quality and availability, selecting the right vendors to collaborate with, and investing in the necessary infrastructure and technology; solutions include collecting and organizing data, selecting appropriate AI algorithms, choosing AI technologies that align with company goals and needs, testing and validating systems before implementation.
Data Quality And Availability
Data quality and availability are significant challenges in implementing AI in supply chains. Organizations need to locate, consolidate, and analyze existing data effectively to maximize the benefits of AI-powered supply chain management.
However, poor data quality or inconsistent inputs can negatively impact forecasting accuracy or predictive modeling.
According to a survey by BCG, supply chain leaders have not been able to effectively harness the power of AI due to data-related issues. One solution is investing in data cleaning and validation procedures before integrating AI technologies into existing systems.
This approach helps ensure that relevant datasets are complete, accurate, and reliable for optimal decision-making processes.
Infrastructure And Technology
One of the main challenges in implementing AI in supply chains is having the proper infrastructure and technology to support it. This includes hardware, software, and network capabilities to handle large amounts of data and power AI algorithms.
Investing in modern technologies such as IoT devices, ERP systems, and warehouse management software can help facilitate smoother communication between different areas of the supply chain and provide valuable insights into operations.
According to McKinsey & Company, companies that invest heavily in digital transformation can achieve up to 3 times higher EBIT growth than their peers who lag behind.
Vendor Choice And Collaboration
Selecting reliable and trustworthy partners is essential to overcome the challenges of implementing AI in supply chains. The choice of a vendor plays a significant role in the success of AI implementation in supply chains.
Effective collaboration and communication routes should be maintained between vendors and other stakeholders in the AI implementation process. Organizations can invest in data cleaning and validation procedures to address the challenges associated with implementing AI.
A thorough technology audit is recommended to identify and address infrastructure and technology-related challenges.
Preparing Your Supply Chain For AI Integration
Establish clear goals and collect and organize data to prepare for AI integration in your supply chain.
Establish Goals
Before integrating AI into the supply chain, it’s crucial to establish goals for what you want to achieve with this technology. The goals should align with your overall business strategy and address specific pain points in your supply chain operations.
By establishing concrete objectives upfront, you can better evaluate the success of your AI implementation and measure its ROI. Furthermore, having a clear sense of purpose will help guide decision-making as you select technologies and develop data models that work best for your needs.
Collect And Organize Data
Collecting and organizing data is vital when integrating AI capabilities into existing supply chain systems. Companies must gather data from various sources, including customer demand patterns, inventory levels, freight volumes, and transportation routes.
The harvested information should be as extensive and detailed as possible to ensure accurate predictions by the AI models.
One example of how data collection has helped companies improve their supply chains comes from Zebra Technologies. They use IoT devices to monitor trucks’ loading processes, helping them optimize truck scheduling and reduce costs associated with carrying excess inventory.
Data Preparation And Cleaning
To effectively integrate AI into supply chain management, data preparation, and cleaning are necessary steps. AI algorithms require high-quality data to produce accurate predictions and insights.
If a company aims to use AI for demand forecasting, it must ensure that the collected data includes relevant factors like historical sales trends, seasonal fluctuations in demand, pricing changes, marketing campaigns, and customer feedback.
Any missing or incomplete information must be filled in before using the algorithm. Additionally, any duplicate or irrelevant data should be eliminated to maintain accuracy.
AI Algorithm Selection
One of the critical steps in preparing your supply chain for AI integration is selecting the right AI algorithm. For instance, descriptive analytics are great for identifying trends from historical data, while prescriptive analytics can provide actionable insights on optimizing results.
Another example is cognitive analytics which can help detect anomalies or identify patterns within unstructured data sets.
McKinsey & Company predicts that AI adoption in the supply chain will grow significantly between 2022 to 2025. Taking the time needed for proper research and testing is crucial before deciding on an algorithm that meets your specific needs.
Choose AI Technologies
Once you have established your goals and collected and organized data, it’s time to choose the AI technologies that will optimize your supply chain. Various AI tools can help with different aspects of your supply chain management, such as demand planning, inventory optimization, and route optimization.
It’s crucial to consider your business’s specific needs when deciding which AI technologies to integrate into your existing systems. Some popular options include machine learning (ML) algorithms for predictive maintenance and demand forecasting; robotic process automation (RPA) for back-office automation; digital twins for real-time insights into operations; optical character recognition (OCR), data entry automation, and other similar tools for automatic data collection and processing.
Data Modeling
Data modeling plays a crucial role in integrating AI into supply chain optimization. Essentially, it involves creating a simplified representation of data that makes it easier to analyze and interpret.
In this case, data modeling is used to help AI algorithms identify patterns within large amounts of supply chain data, such as sales figures, inventory levels, and delivery schedules.
Moreover, an accurate model will enable companies to plan better for future challenges by predicting potential bottlenecks or delays in the supply chain. It also helps them identify areas where efficiencies could be gained through automation or other improvements.
Of course, constructing these models correctly requires high-quality data from multiple sources, along with specialized software tools capable of handling complex datasets with ease and accuracy.
Integrate With Existing Systems
Integrating AI into a supply chain involves linking various systems and platforms such as Enterprise Resource Planning (ERP), Warehouse Management System (WMS), and Transportation Management System (TMS).
This requires constructing features that explain the variability in the data and ensuring seamless communication between different departments. For instance, AI-based demand forecasting tools can be integrated with inventory management systems to optimize stock levels based on predicted demand.
Successful integration of AI algorithms requires thorough testing and validation before deploying solutions across the entire supply chain network. It also necessitates continuous improvement efforts for optimal productivity, accuracy, and decision-making performance.
Investing in reliable data visual analytics platforms powered by AI is critical to minimizing supply chain disruptions while maximizing business outcomes.
Test And Validate
Thorough testing and validation of AI models and linked systems are critical to ensure their precision, dependability, and performance when integrating AI into the supply chain.
Companies must pilot test and deploy on a smaller scale before implementing AI solutions across the entire supply chain to evaluate the AI system’s performance and make necessary improvements.
For example, in 2014, retail giant Walmart implemented an AI-based demand sensing tool that used store point-of-sale data to accurately forecast customer purchases.
However, they started with a limited rollout in one region before expanding it nationwide after validating its effectiveness.
Repeated testing is essential as it ensures that the integrated technology runs seamlessly throughout different development phases without negatively affecting current operations.
The Future Of AI For Supply Chain Management
Advancements in technology are driving AI technologies that can process high amounts of data and provide real-time insights into global supply chains.
Additionally, machine learning algorithms are becoming increasingly sophisticated, enabling prediction and analysis models that optimize supplier management and inventory control further.
The potential benefits of integrating AI into supply chain operations are clear: reduced operating costs, increased efficiency across route optimization and demand forecasting processes, and greater scalability through automation capabilities such as warehouse robotics or automated quality checks.
In conclusion – Deployment of AI-powered solutions will improve efficiencies within warehouses; streamline transportation routes; support predictive maintenance schedules for fleets which reduces downtime while enhancing asset performance visibility for decision-makers responsible for operational planning leading to fewer disruptions overall throughout all aspects associated with managing effective end-to-end supply chains optimizing processes continuously where possible thanks in part due advancements made over time by new vendors entering markets globally who specialize exclusively around developing specialized software packages tailored specifically intended purpose ensuring maximum ROI against investment made evaluating functionality features provided when making selections about which systems should be adopted going forward based on unique use cases identified at individual companies aiming achieve competitive differentiation among peers competing industry verticals looking transform digitally utilizing advances offered by fourth industrial revolution enabled smart factories using IoT sensors connected networks leveraging cloud-based processing power analyzing vast datasets automatically delivering actionable insights aiding operators’ decision-making capabilities allowing them prepare proactively meet challenges ahead staying agile nimble dynamically responding market conditions changes providing greater flexibility meeting rapidly changing customer demands.
Conclusion
AI is transforming the world of supply chain management by providing unparalleled insights and optimization. With AI-enabled tools such as predictive analytics and automation, businesses can reduce costs, improve sustainability efforts, and enhance supplier relationships.
Despite data quality and infrastructure challenges, companies can prepare for AI integration by setting goals, collecting and organizing data, and selecting appropriate technologies and algorithms while integrating with existing systems to maximize benefits.
The future of AI in supply chains is promising, with continued innovation around digital transformation initiatives that leverage IoT devices to provide real-time tracking mechanisms that ensure on-time delivery while minimizing operational costs.
FAQ
How Does AI Help Supply Chain Optimization?
AI plays a significant role in optimizing supply chains by automating and streamlining complex processes. Machine learning algorithms help reduce operational costs by identifying and predicting bottlenecks, optimizing routes, and improving on-time delivery.
AI-powered predictive analytics and forecasting tools enable companies to track inventory levels accurately, identify demand fluctuations, prevent stockouts, and make informed business decisions based on region-specific data.
Additionally, AI enhances supplier relationship management by providing real-time updates on product availability while ensuring sustainability goals are met through carbon emissions reduction initiatives such as greener transport logistics and warehousing.
What Is AI Strategy For Supply Chain?
AI strategy for the supply chain is the deliberate and systematic plan of integrating artificial intelligence technologies to improve operational efficiency, reduce costs, enhance customer service, and increase profitability.
Companies that have successfully adopted AI in their supply chain management develop clear objectives, select suitable algorithms for data modeling, integrate new technology with existing systems, and test and validate results before implementation.
For instance, logistics companies use AI-powered routing optimization software to determine the fastest delivery route while minimizing fuel usage.
How Can AI Solve Supply Chain Problems?
AI can solve several supply chain problems, from improving inventory management to optimizing transportation routes and enhancing supplier communications. AI-powered predictive analytics can help prevent stockouts by accurately forecasting demand, while automated quality checks can improve product consistency and reduce waste.
Furthermore, AI tools such as natural language processing (NLP) and computer vision enable better supplier communication through efficient document processing and verification.
These technologies allow supply chain managers to track shipments, monitor inventory levels, streamline warehouse operations, optimize production schedules, and minimize shipping times.
How Can AI And Analytics Optimize Supply Chain?
AI and analytics can optimize the supply chain by giving businesses real-time visibility into their operations, enabling them to make informed decisions. For instance, predictive analytics leverages historical data and machine learning algorithms to forecast future demand, reducing stockouts and improving customer satisfaction.
Moreover, AI-powered chatbots automate supplier communications while natural language processing (NLP) enables businesses to extract insights from unstructured data such as customer feedback.
By integrating these tools into their supply chain processes, companies can significantly reduce the time spent on manual tasks while improving accuracy and efficiency.
How AI Can Make Supply Chains More Sustainable?
AI has the potential to make supply chains more sustainable and eco-friendly. By leveraging predictive analytics, AI-powered tools can optimize transportation routes, reducing emissions and miles traveled.
This reduces the carbon footprint of the supply chain while cutting operational costs. Additionally, accurate inventory management through AI can prevent overstocking and inadequate stocking, reducing waste.
The big data generated by AI technologies can help supply chains become sustainable and resilient in times of disruption, such as natural disasters or pandemics.
Data indicates that adopting new digital technology solutions like AI will go a long way toward driving sustainability initiatives within the logistics industry: nearly two-thirds (63%) of shippers surveyed said increased use of technology would be critical to meeting their sustainability goals over the next five years; almost half (48%) ranked hyper-scale computing infrastructure among their top-three tech investments needed for making progress towards those objectives.
What Is The Disadvantage Of AI In the Supply Chain?
One major disadvantage of using AI in supply chain management is the potential displacement of human workers. As more tasks become automated through AI-operated systems, certain job functions may no longer require human intervention.
Another consideration when implementing AI-powered solutions in the supply chain is the high cost associated with maintaining and replacing parts for these advanced systems.
These expenses can add up quickly over time, making it important for companies to carefully weigh the benefits against costs before fully embracing this technology.
Will AI Replace Supply Chain Management?
AI will not replace supply chain management but instead assist and improve it. Supply chains are complex and require human decision-making skills to handle unforeseen situations.
AI can help with tasks like forecasting, inventory optimization, quality control, and logistics automation to make the process more efficient and cost-effective. While AI can efficiently crunch data and provide insights into trends, humans still have a crucial role in managing supplier relationships and making critical business decisions.
AI offers significant advantages in cost savings, risk mitigation, and improved forecasting accuracy that benefits supply chain operations but doesn’t mean it will eliminate jobs completely.
In fact “Gartner” predicts that by 2023 only one-third of global logistics will utilize any level of automation involving robotics or autonomous vehicles.
How can AI be used for supply chain optimization?
AI can analyze high amounts of data to identify patterns and optimize supply chain operations, such as inventory management, production scheduling, and transportation routes. It can also predict real-time demand changes and potential disruptions, enabling companies to make proactive decisions.
What benefits does using AI bring to supply chain management?
Some benefits of using AI include improved efficiency, reduced costs, increased accuracy in forecasting demand, and minimizing the risk of errors in manual processes. Additionally, it provides a competitive advantage by allowing companies to react quickly to changes in the market.
Do I need specialized knowledge to use AI for supply chain optimization?
While having some technical knowledge is helpful when implementing an AI solution into your business strategy, many third-party providers offer user-friendly software that requires minimal coding skills or IT expertise.
Is investing in AI solutions financially feasible for small businesses?
The cost of implementation depends on various factors such as the complexity of the system required; however, there are several affordable options available at different levels depending upon resources available – especially if partnering with third-party services who specialize specifically within this area (i.e., startups focused exclusively on creating software designed around helping small-to-medium sized enterprises reap the rewards delivered through automation).