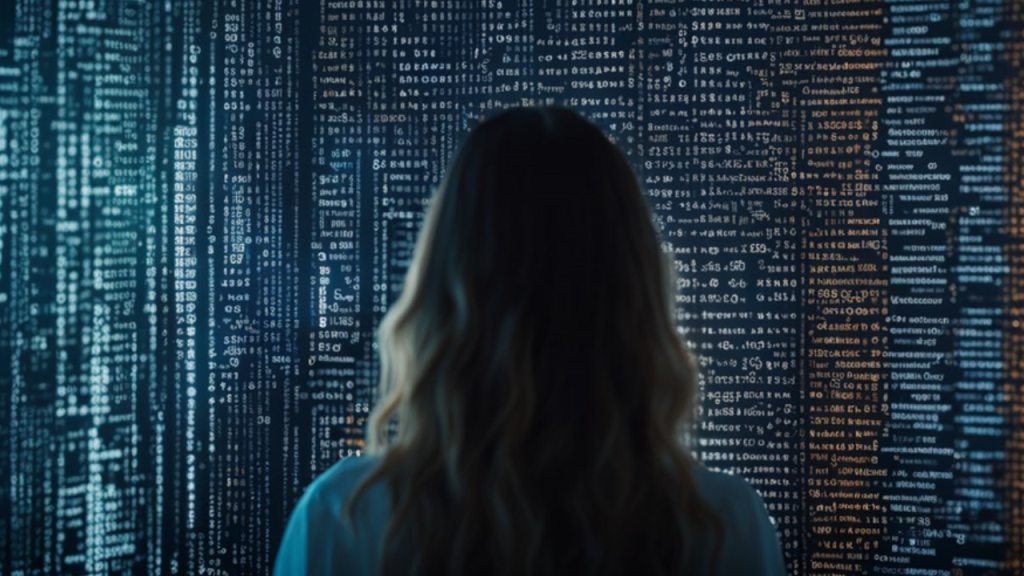
Today’s digital landscape is brimming with potential threats that demand high vigilance. The advent of Natural Language Processing (NLP) has revolutionized the cybersecurity field, unearthing a powerful tool for identifying and mitigating these threats.
This article will guide you through how NLP works, its benefits to cybersecurity, and notable ethical considerations. Ready? Let’s dive into this captivating realm of technology!
Key Takeaways
- Natural Language Processing (NLP) helps machines understand and reply to human language, but this comes with challenges.
- NLP can transform cybersecurity by finding threats quickly and effectively.
- There are ethical concerns like bias in NLP, which must be carefully handled.
- Integrating NLP into cybersecurity improves protection but raises complex privacy questions.
Understanding Natural Language Processing (NLP)
Natural Language Processing (NLP) is a revolutionary aspect of Artificial Intelligence that enables machines to comprehend, interpret, and respond to human language. It faces immense complexities due to the nuances and subtleties inherent in human language.
Moreover, ethical considerations are key if NLP tools are to be deployed responsibly. Despite these challenges, NLP has incredible potential for revolutionizing cybersecurity by efficiently and effectively identifying and combating cyber threats efficiently and effectively.
The challenge of human language
In all its complexity, human language presents a significant challenge for artificial intelligence and machine learning. It’s rich in nuance, idiomatic expressions, cultural references, and regional variances – elements that are not easy for AI systems to grasp fully.
To add to this complexity, human communication goes beyond just the words spoken or written; it also involves context. The same word can have varied meanings based on the situation or speaker’s intent.
Processing this intricate web of linguistic variables demands advanced language-modeling tools capable of recognizing patterns and predicting outcomes accurately. Natural Language Processing (NLP) technologies utilize unsupervised AI models like word embeddings to tackle this issue head-on by capturing underlying biases within these datasets.
However, if unchecked, these biases can proliferate across downstream applications, leading them towards biased decisions, thus perpetuating historical prejudices and inequities. Current technological capabilities haven’t yet mastered regulating such potential threats imposed by AI technologies onto principles of justice, equity, and democracy.
Ethical Considerations for NLP
Effective natural language processing (NLP) presents ethical implications that warrant careful attention. Often, NLP implementations rely on artificial intelligence models like word embeddings, which can inherently harbor biases extracted from the language data they process.
This implies an intertwining of AI and bias – a concerning fact for manufacturers and users alike.
These biases often mirror societal divisions and prejudices, including gender or race. For instance, racial bias in NLP can fuel discrimination against specific social groups, bordering on violating data privacy laws.
Similarly, gender bias in NLP can present deceptive results, skewing job candidate selection unfairly towards one sex.
Policymakers must establish regulatory mechanisms cooperating with businesses utilizing these technologies to ensure fairness in Natural Language Processing systems. Such regulations should prioritize auditing AI technologies alongside stringent compliance standards, ensuring objectivity prevails within their algorithms’ decision-making processes.
The Intersection of NLP and Cybersecurity
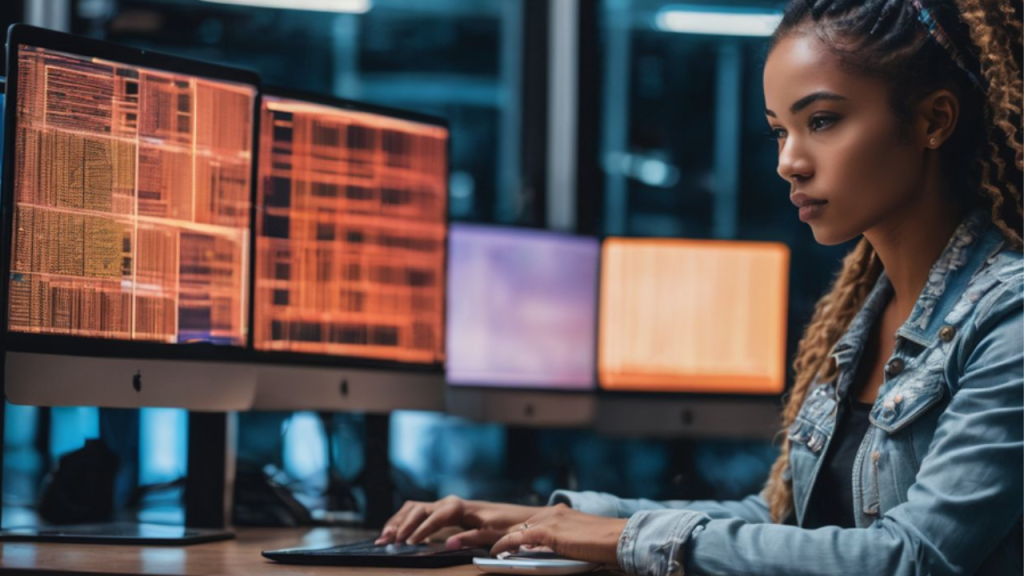
Natural Language Processing (NLP) plays a crucial role in the dynamic sphere of cybersecurity. Experts are finding that NLP significantly transforms cyber risk and compliance management with its ability to decode complex human language into computing algorithms.
Being adept at identifying and mitigating cyber threats makes NLP an essential tool in contemporary cybersecurity strategies. Despite being beneficial, integrating NLP with cybersecurity comes with challenges that tech specialists need to address for seamless operations.
How NLP is transforming cyber risk and compliance
Natural Language Processing is gradually revolutionizing how businesses manage cyber risk and ensure compliance. NLP, a subfield of artificial intelligence, focuses on the interaction between human language and machines.
In recent years, it has emerged as a robust ally in cybersecurity efforts due to its ability to comprehend, interpret, and utilize data from various sources, including emails, chat logs, and customer feedback.
A key advantage provided by Natural Language Processing lies in its capacity for real-time detection of potential breaches or threats. Applying machine learning algorithms enables companies to identify anomalies quickly through text-based channels such as email messages or social media posts.
Another area where NLP plays an essential role is enhancing incident reporting capabilities, which can analyze large volumes of past incident reports to uncover patterns that may elude manual analysis.
Thereby allowing cybersecurity professionals to proactively address situations before they escalate into serious issues.
NLP’s role in identifying and mitigating cybersecurity threats
Natural Language Processing (NLP) is gaining ground as an essential tool in the cybersecurity landscape. It uses advanced AI models and machine learning algorithms to analyze text data from diverse sources, including emails, chat logs, and social media posts.
NLP can sift through vast amounts of information quickly and efficiently to detect patterns that indicate potential cyber threats.
By classifying malicious messages or flagging unusual activity in real-time, NLP technology allows security teams to act swiftly against possible breaches. Not only does it identify current risks, but it also anticipates future threats by analyzing trends and patterns hidden within the data.
In today’s digital era, where harmful software such as phishing attacks operate primarily via textual communication – effective language analysis carried out by NLP tools can result in significantly more robust digital protection for individuals and organizations.
Using sophisticated models like GPT-3, certain Natural Language Processing applications can detect even nuanced forms of threat-related text in customer communications or incident reporting systems.
Additionally, integrating NLP with existing data privacy laws enhances its capacity to identify risk and ensures proper governance compliance alongside improved data protection initiatives across all business spheres.
This integration enables a proactive approach for organizations seeking strong cybersecurity measures while enriching their IT asset inventory management solutions.
The benefits and challenges of integrating NLP and cybersecurity
Integrating NLP into cybersecurity comes with immense benefits, but it’s not without challenges. The main advantage lies in NLP’s ability to analyze text and language, making sense of unstructured data like chat logs or emails.
This allows for real-time detection of potential breaches and quick responses to phishing attacks. By leveraging machine learning algorithms and automated customer services through chatbots, organizations can proactively address issues, providing better protection overall.
However, the intersection of NLP and cybersecurity isn’t seamless; a single bias in AI models used by NLP could throw off an entire system’s accuracy. Specifically, biased decision-making due to racial or gender bias baked into word embeddings can cloud judgment, leading to counterproductive outcomes rather than enhancing security measures.
Another major hurdle is that privacy implications become increasingly complex as AI technologies delve deeper into human language understanding; considering HIPAA regulations within the healthcare sector, data encryption becomes critical when using intelligent tools that deal directly with customers’ personal information.
Bias in Natural Language Processing
Despite the advancements in Natural Language Processing (NLP), there exists an undeniable issue of bias within its system, such as biases evident in word embeddings. On a larger scale, gender and racial biases surface and pose challenges to fairness even as researchers grapple with the problems associated with debiasing based on social group associations.
Biases in word embeddings
Natural Language Processing (NLP) utilizes word embeddings to extract meaning from human language. Despite their powerful potential, biases can infiltrate these models, potentially perpetuating historical injustices and societal prejudices within AI technologies.
The impact of such biases extends beyond theoretical discussions, as biased decisions made by NLP applications may result in discriminatory behavior.
Word embeddings often mirror existing social biases within the datasets they are trained on. This is evident when examining co-occurrences in word relationships. Names associated with African Americans tend to link with unpleasant words more frequently than others, reflecting a deep-seated racial bias pervading AI models.
Even more concerning is how the intersectionality of race and gender further exacerbates bias within word embeddings. An example lies in state-of-the-art language models where men correlate more frequently with competency and higher education levels. At the same time, women align closer to family terminology and literature themes.
Herein lie worrisome implications against specific social groups, particularly minority ones like African American women who face dual discrimination based on ethnicity and gender boundaries entrenched into the biased language data used for training these machine learning algorithms.
Racial and gender bias in NLP
Racial and gender biases persist in Natural Language Processing (NLP). Using AI technologies like unsupervised NLP models might inadvertently promote harmful stereotypes because these AI models mirror human faults.
Certain language corpora machine learning algorithms used to understand human speech have shown inherently skewed tendencies. These biased decisions lead to further inequalities in society.
Strong evidence has been found that showcases the existence of racial bias in word embeddings, a crucial building block for many advanced NLP tasks such as generating chatbots or analyzing text and language.
For instance, African-American names frequently co-occur with negative words within these supposedly unbiased databases, indicating clear prejudice.
Similarly, large language models like GPT-3 are attributed to an established gender bias they picked up during their training process from data sources dominated by biased human-generated text.
Advanced business functions depending heavily on customer communications via emails or chat logs could be significantly impacted if they leverage AI tools containing sexist views learned from historical data patterns.
Summaries generated through biased articulations can potentially associate men with competence and higher education. In contrast, women are associated unjustly with family roles and literature topics, leading to substantial concerns around fairness in how our digital technology progresses.
The problems of debiasing by social group associations
Unsupervised AI models tend to mirror the biases found in their training data, exacerbating issues of discrimination and bias. They often connect words representing social groups with negative or positive attributes based on historical usage patterns.
For instance, African-American names are regularly associated with unpleasant terms within word embeddings. This correlation reflects harmful preconceived notions that can continue if left unchecked in Natural Language Processing algorithms.
Attempting to debase these systems presents a tricky challenge as it involves making assumptions about the fairness of certain associations over others. These decisions can inadvertently favor one group while further marginalizing another.
Furthermore, neutralizing these biases does not fully solve the issue as vital distinctions between demographic groups may be irresponsibly eliminated in the process––complicating rather than resolving matters of fairness and representation in NLP technology.
Enhancing Cybersecurity with NLP
Explore how Natural Language Processing can amplify cybersecurity measures, making threat detection more immediate and response swift. Uncover the benefits and best practices of wielding NLP in your cyber defense strategy – keep reading for a deep dive into this cutting-edge evolution in digital security.
Use of NLP for cybersecurity awareness and education
Nurturing a well-informed team is integral for cybersecurity. Leveraging Natural Language Processing (NLP) is an efficient strategy to achieve this goal. This AI-based technology enables the development of personalized cybersecurity training programs that use real-world scenarios and examples relevant to an individual’s roles and responsibilities.
Natural Language Processing can sift through massive amounts of data, including chat logs, emails, and incident reports. Identifying patterns hidden in these sources allows for not just threat detection but also an understanding of common pitfalls and preventative measures.
This analysis empowers organizations with knowledge of potential breaches or vulnerabilities tied to human error.
In addition, NLP plays a crucial role in equipping teams with apt tools for maintaining robust security practices. These tools can predict phishing attempts or detect malicious code embedded within innocuous-looking text prompts.
Propelling our workforce toward higher security consciousness becomes less challenging when aided by the insights derived from NLP applications.
Best practices and considerations for developing secure NLP solutions
Designing secure NLP solutions entails a broad range of considerations. A robust approach to curtail the risk of bias is indispensable when dealing with unsupervised AI models like word embeddings, which have been observed to propagate biases onto downstream applications.
Regular audits and reviews for language corpora mitigate these risks, reducing potential unpleasant associations such as racial and gender biases detected in earlier studies.
Additionally, giving due attention to data privacy laws should be a priority given the sensitive nature of data involved in NLP applications; these could range from health records in healthcare sector programming to chat logs implemented in customer service bots.
Balancing regulations like HIPAA or GDPR against the need for well-populated databases isn’t merely standard compliance – it also ensures that your solution respects individual rights while maintaining its functionality.
Encryption is a good practice, too, as it protects information during transactional processes and storage.
Policy Measures for Fairness in NLP
Policymakers can play a pivotal role in the quest for fairness in NLP by establishing guidelines and regulatory mechanisms that help mitigate biases, striking a balance between innovation and safeguards.
The detailed exploration of these measures makes an intriguing read – find out more!
What can policymakers do to create fairness in NLP?
Policymakers can take several steps to ensure fairness in Natural Language Processing (NLP). First, they could establish regulations to audit AI technologies. Regular audits would detect potential threats and any violations of equity norms early, allowing for corrective measures.
Besides auditing, introducing guidelines specifically concerning AI and NLP is essential. These guidelines need to pay attention to the ethical use and address bias implications for gender, race, and age.
Creating legal frameworks that monitor NLP applications should be another priority for policymakers. Enforcing strict penalties against biased decisions propagated by these systems will foster more accountable practices among developers and businesses.
In addition, setting up transparent regulatory mechanisms discourages unethical uses while reassuring users about their privacy and security when interacting with AI models.
Lastly, implementation strategies vouching for diversity during the data collection phase within AI training models should be pushed forward by policymakers. This approach provides a broader representation of societal nuances, thus reducing inherent prejudice that might otherwise seep into algorithms during machine learning processes.
Conclusion
“The power of Natural Language Processing revolutionizes cybersecurity, offering innovative solutions for threat identification and mitigation. However, the bias inherent in NLP challenges ethical principles and fairness. With effective policy measures and fair practices in place, we can harness the full potential of NLP while ensuring its safe use.