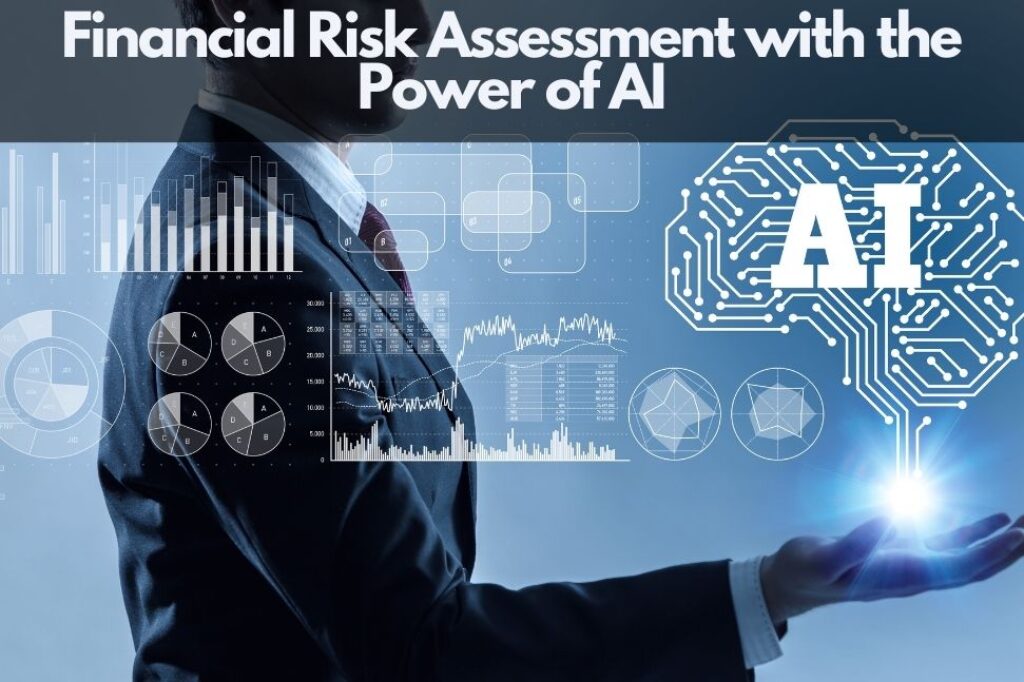
Artificial Intelligence (AI) is transforming how we assess and manage these risks by harnessing the power of algorithms and big data analytics. Here we’ll explore how cutting-edge AI technologies are reshaping financial risk assessment to increase accuracy, efficiency, and agility in decision-making.
Table of Contents
Key Takeaways
- Financial institutions face a complex landscape of risks, but leveraging AI and machine learning can help improve accuracy, efficiency, and agility in decision-making.
- Key use cases of AI in risk management include predictive and prescriptive analytics for identifying patterns, root cause analysis to determine underlying causes, and risk intelligence for detecting early warning signs of potential risks.
- Benefits of using AI for financial risk assessment include improved accuracy in detecting potential risks such as non-payment or fraudulent activity, faster adaptation to new risks through real-time data analysis and automated processes, and cost reduction by optimizing resource allocation.
Importance Of Risk Assessment In Finance
Risk assessment is a critical aspect of the financial industry, as it helps institutions manage and mitigate potential threats that could compromise their stability and profitability.
It involves identifying, analyzing, and evaluating various types of risks, such as credit risk, fraud risk, operational risk, market risk, liquidity risk, cybersecurity risk, and reputational risk.
One prime example of financial risk assessment’s significance is loan underwriting. Lenders must accurately evaluate the creditworthiness of applicants to minimize non-payment issues and maintain a healthy portfolio.
An inaccurate evaluation can lead to increased defaults resulting in financial loss and reputation damage for lenders. Additionally, banks face regulatory scrutiny, which requires them to meet specific capital requirements tied to their overall exposure level across diverse asset classes.
Financial institutions also need effective fraud detection systems since they are frequently targeted by criminals seeking to exploit vulnerabilities in transactions for personal gain – especially true with the surge in digital banking services used today.
In this context, accurate assessment becomes vital for minimizing customer trust erosion and staying compliant with anti-fraud legal mandates like Anti-Money Laundering (AML) rules or Bank Secrecy Act (BSA).
Role Of Artificial Intelligence In Financial Risk Assessment
Artificial Intelligence plays a vital role in financial risk assessment, from intelligent algorithms for risk management to automating claims and policy processes.
Intelligent Algorithms For Risk Management
Intelligent algorithms for risk management are transforming the finance industry by leveraging AI and machine learning techniques to assess and mitigate risks more efficiently.
For instance, AI-powered credit risk management tools inspect borrower credit history and identify early warning signs of non-payment. Inscribe’s intelligent document automation solution is another example that helps banks manage risk while offering insights into customer creditworthiness.
Automating Claims And Policy Processes
AI-driven automation has revolutionized how financial institutions handle claims and policy processes, resulting in increased efficiency and reduced operational costs.
For instance, Inscribe’s AI-powered fraud detection system helps identify early warning signs of potentially fraudulent activities by scanning documents such as invoices, contracts, or insurance policies.
Additionally, Ventiv Technology offers solutions like Ventiv Claims that expedite the claims management process by automating data entry and validation tasks. This reduces manual errors and enables organizations to focus on value-added services for their customers.
Key Use Cases Of AI In Risk Management
AI is used for predictive and prescriptive analytics to identify patterns, predict future risks, and prescribe mitigation strategies.
Predictive And Prescriptive Analytics
AI can leverage predictive and prescriptive analytics to enable financial institutions to identify potential risks by analyzing vast amounts of data. Predictive analytics uses machine learning algorithms to forecast, while prescriptive analytics provides insights based on those predictions.
For example, AI-powered credit scoring models can use consumer credit histories and other factors to generate real-time risk assessments that support decision-making.
Similarly, banks can use big data analysis for market risk assessment, tracking fluctuation patterns and trends for improved investment strategy development. Additionally, AI helps with stress testing, which is crucial in identifying vulnerabilities in times of crisis.
Also, aiding compliance efforts through predictive monitoring for fraud detection or regulatory noncompliance, thus leading towards cost reduction while speeding up reviews and feedback loop identification.
Root Cause Analysis
Root cause analysis is a crucial AI-powered tool used in financial risk assessment to accurately identify the underlying cause of potential risks. It involves analyzing all factors contributing to a specific risk event, including people, processes, and systems, and determining the core reason that led to that event.
For instance, let’s consider credit risk management. Data analytics-enabled algorithms can help banks better understand their customers’ spending habits and make more informed lending decisions by detecting patterns of high-risk behavior.
Root cause analysis can determine reasons behind non-payment issues, such as insufficient credit score or unexpected income loss related to various risk categories like fraud or cyber threats.
Risk Intelligence
Risk intelligence is essential to financial risk assessment, allowing organizations to better identify and understand potential risks. Utilizing AI technologies such as machine learning and natural language processing, risk managers can analyze data from various sources to gain real-time insights into market factors and detect early warning signs of non-payment or fraudulent activity.
In addition, AI-powered systems enable personalized notifications for mitigating identified risks to help avoid surprise financial losses while automating monotonous processes like monitoring risk indicators.
This results in more efficient resource allocation, helping the organization save on costs over time.
Benefits Of Leveraging AI In Financial Risk Assessment
AI-powered financial risk assessment offers improved accuracy, faster adaptation to new risks, enhanced efficiency, and cost reduction for the banking industry.
Improved Accuracy
By leveraging AI in financial risk assessment, there is a significant improvement in the accuracy of detecting and managing potential risks. In addition, banks can gain better insights into customer behavior and creditworthiness through predictive analytics and machine learning algorithms.
For instance, AI-powered credit risk management tools can detect early warning signs of non-payment by analyzing vast amounts of data points such as past payment history, current account balance, and employment status.
This helps mitigate credit risk for banks.
Additionally, AI-based fraud detection systems can accurately monitor transactions for red flags that may indicate fraudulent activity.
Inscribing AI solutions use behavioral analysis to offer automated fraud checks that compare an individual’s spending habits against his or her typical patterns over time; if there are any anomalies discovered within the usual pattern range – such as larger purchases abroad or through new merchants – it automatically blocks payments until reviewed manually. It has been demonstrated that Inscribing an AI-powered solution being 96% accurate in identifying risky spending, is a great example of improving accuracy using technology.
Faster Adaptation To New Risks
Leveraging AI in financial risk assessment enables organizations to adapt quickly to new risks, reducing the impact of emerging threats. Traditional risk management systems rely on historical data, which may not apply to newer risks.
For instance, in the case of loan underwriting, banks can use AI-powered credit risk modeling tools that consider the borrower’s digital footprint and behavior patterns.
This approach helps identify potential defaulters even before they miss a payment while mitigating losses for the bank.
By leveraging intelligent automation and big data analytics capabilities with robust algorithms that provide actionable insights and analysis in real-time such as Inscribe’s AI-powered fraud detection or root cause analysis solutions offered by other providers- businesses can stay ahead of emerging trends making strategic decisions with confidence while understanding any associated risks better.
Enhanced Efficiency
Implementing AI-driven risk management solutions can enhance efficiency in financial risk assessment. With AI technologies, data is processed much faster, accelerating analysis and decision-making processes.
Furthermore, implementing AI-powered fraud detection solutions helps reduce costs associated with cybercrimes while strengthening cybersecurity protocols. By automating process flows for operational efficiency, financial institutions can prevent investment issues that arise from inconsistent or faulty procedures.
Cost Reduction
Leveraging AI for financial risk assessment can lead to significant cost reduction. By automating and streamlining processes, banks and other financial institutions can save time and money while improving accuracy.
For example, AI-powered fraud detection systems can quickly analyze vast amounts of data to detect any suspicious activity, preventing potential losses for the company.
AI-fueled solutions can also accelerate compliance procedures, reducing costs and preventing further money loss. For example, NLP technology allows the analysis of millions of regulatory data to enable financial service providers to understand changes and implement immediate requirements without going through lengthy manual operations or hiring additional staff.
Furthermore, structural and mindset changes are necessary for industry leaders looking to leverage multi-dimensional AI capabilities across their organizations fully.
Implementing AI-Driven Risk Management Solutions
To ensure the successful implementation of AI-driven risk management solutions, it is crucial to choose the right AI/ML solution and integrate it seamlessly into existing risk management systems – read on to learn more about how AI can transform financial risk assessment!
Choosing The Right AI/ML Solution
Selecting the right AI and machine learning (ML) solution is essential to implementing AI-driven risk management solutions in the finance industry. There are several factors that banks and financial institutions must consider when choosing an AI/ML solution, including its compatibility with existing systems, data quality, stress testing capabilities, early warning systems for potential risks, personal assistant features, and digital labor capabilities.
Automated solutions leveraging AI/ML can enable banks to streamline processes while improving their ability to quickly understand and respond to risks.
Integrating AI Into Existing Risk Management Systems
AI can be integrated into existing risk management systems to enhance their capabilities and provide more accurate predictions of potential risks. This can be achieved through the following ways:
- Data Integration: Bringing data from different sources together and integrating them with AI algorithms to identify patterns and trends that may be relevant for risk assessment.
- Risk Modeling: Use AI-powered risk modeling tools to analyze data and create predictive models that improve decision-making related to risk management.
- Fraud Detection: Incorporate AI-powered fraud detection tools into existing systems to help detect fraudulent activities in real time, minimizing financial losses.
- Cybersecurity: Improve cybersecurity by using AI to monitor networks and detect suspicious activity, making it easier to identify potential threats before they cause any damage.
- Compliance Management: Automate regulatory compliance processes using artificial Intelligence, such as monitoring compliance with legal regulations or preventing money laundering activities.
- Root Cause Analysis: Utilize AI-powered root cause analysis tools that examine risk factors across systems and uncover insights into complex issues underlying risks.
By integrating AI into existing risk management systems, businesses can benefit from better accuracy, faster adaptation to new risks, enhanced efficiency, and reduced costs associated with managing risk over time.
How AI Can Be Used In Risk Management?
Artificial Intelligence (AI) offers several applications in risk management. With big data analytics, AI systems can identify patterns and trends that may not be apparent to human analysts, enabling earlier detection of potential risks.
For instance, loan underwriting is an excellent example of how AI can streamline the credit approval process while mitigating risks.
Similarly, AI-powered fraud detection helps prevent financial crimes such as insider trading by monitoring trader activity to detect early signs of fraudulent behavior.
Implementing AI-driven solutions into existing risk management practices helps organizations reduce operational costs associated with manual processes while improving accuracy levels in identifying threats that would have otherwise been missed using traditional methods.
Which Is An Example Of AI That Would Help Management Accountants And Financial Analysts?
AI can be used in various ways to help management accountants and financial analysts. One example is using natural language processing (NLP) to extract data from documents such as financial statements, tax returns, and invoices.
This helps automate the data entry process and frees up time for analysts to focus on higher-level tasks such as forecasting and analysis.
Another example is applying machine learning algorithms for risk modeling and fraud detection. By analyzing large volumes of historical data, AI systems can identify patterns that indicate risky behavior or potentially fraudulent activity.
Overall, the use of AI in finance has revolutionized how accountants and analysts approach their work by enabling faster decision-making, improved accuracy, enhanced efficiency, cost reduction, and better compliance management with national regulations, among other benefits listed in [IMPORTANT FACTS].
What Is AI In Financial Risk Analysis?
AI in financial risk analysis involves leveraging machine learning algorithms and big data analytics to identify potential risks, trends, and patterns.
For example, AI-powered fraud detection can detect anomalies in transactional data that suggest a fraudulent activity, while credit risk models can help predict loan defaults by analyzing historical payment performance.
By automating identifying potential risks early on, AI helps prevent losses before they happen.
Conclusion
In conclusion, leveraging AI for financial risk assessment transforms the banking industry by improving accuracy, efficiency, and cost reduction. With intelligent algorithms, predictive and prescriptive analytics, root cause analysis, and risk intelligence, banks can effectively manage credit, fraud, operational, market, and cybersecurity risks, among others.
By choosing the right AI/ML solution and integrating it into existing systems, system vulnerabilities can be easily identified while malicious activity is mitigated to protect against cyberattacks.
FAQs
What is AI, and how can it be used for financial risk assessment?
AI, or artificial Intelligence, uses software algorithms to analyze data and make predictions based on patterns identified within that data. For example, in financial risk assessment, AI can analyze large amounts of historical financial information to identify potential investment risks.
Can AI completely replace human analysts in conducting financial risk assessments?
While AI technology has advanced significantly in recent years, it cannot fully replace human expertise when assessing complex financial risks. It is best used as a tool to supplement an analyst’s abilities, providing additional insights and analysis that can help inform decision-making.
How accurate is AI technology for predicting financial risks?
The accuracy of any risk prediction tool depends on the quality of the data being analyzed and the sophistication of the algorithm being used. However, studies have shown that utilizing AI tools for analyzing large datasets provides higher accuracy levels than traditional methods.
What common challenges are associated with implementing AI for financial risk assessment?
Some common challenges include issues around data privacy and security, ensuring transparency in decision-making processes by creating easily understandable explanations for decisions made by an algorithm-based system, and concerns about bias or unfairness in results due to programming errors or incorrect assumptions built into algorithms, among other technical considerations involved in deploying these highly sophisticated systems effectively across different organizational structures or jurisdictions globally.