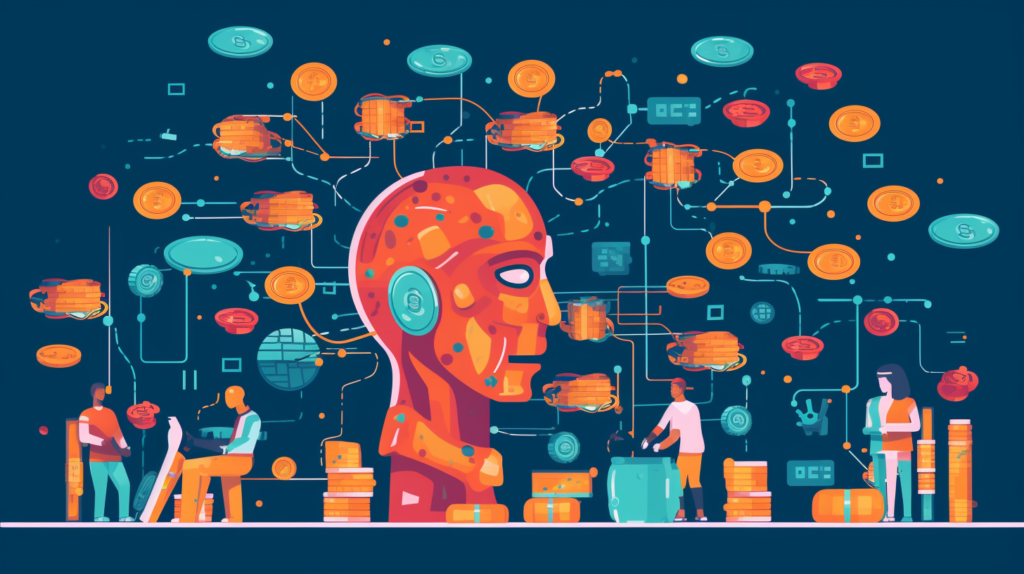
Dynamic pricing is a method used by modern businesses to optimize their pricing strategy and attract more customers while increasing profits. With traditional approaches becoming obsolete, setting the right price has become a significant challenge for many companies. However, with the power of artificial intelligence in dynamic pricing algorithms, businesses can now achieve more precise results through data-driven insights and machine learning capabilities. By implementing different models such as Bayesian, reinforcement learning, or decision tree models with various modules like long tail or elasticities module for optimization purposes, businesses can maximize revenue and profit while minimizing customer churns and aligning them with business objectives. This post explores how AI-optimized pricing strategies work and why they’re essential to success in today’s competitive market.
Table of Contents
Key Takeaways
- Dynamic pricing AI is a powerful tool that allows businesses to optimize their pricing strategies for increased revenues and profits while minimizing customer churn.
- Machine learning is crucial in making intelligent pricing decisions by analyzing vast amounts of historical and real-time data to generate accurate insights.
- Different models and modules are available for implementing dynamic pricing AI, including Bayesian, reinforcement learning, and decision tree models with long tail or elasticities module for optimization purposes.
- By embracing artificial intelligence-powered dynamic pricing strategies, companies can stay ahead of the curve in this rapidly-changing landscape while maintaining satisfied, loyal patrons through personalized offers tailored specifically towards individual needs/preferences tracked over time using advanced analytics techniques (machine learning algorithms).
Traditional Approaches To Pricing
Traditional approaches to pricing can be categorized into three main types: cost-based, competitor-based, and demand-based. In cost-based pricing, businesses calculate the total production costs for a product or service and then add a markup to determine its selling price.
Competitor-based pricing involves setting prices based on competitors’ charges for similar products or services. This approach helps a business stay competitive but might ignore variations in brand value, product quality, or target audience demographics.
Demand-based pricing is driven by consumer demand and perceived value; businesses set their prices according to how much customers are willing to pay while considering seasonal trends and purchasing patterns.
The Challenge Of Setting The Right Price
Setting the right price for products and services is critical to running any successful business. It involves striking a delicate balance between enticing customers with competitive prices and maintaining profitability to sustain operations.
One well-known example of this challenge comes from the retail industry, where stores must constantly adapt their pricing strategies to shift consumer preferences, trends, and competitor activities.
Additionally, seasonal fluctuations and varying demand levels complicate determining appropriate pricing structures.
The Power Of Artificial Intelligence In Dynamic Pricing
AI algorithms can process large amounts of historical and real-time data to provide machine learning predictions, allowing businesses to optimize their pricing strategies at a speed and scale previously impossible with traditional approaches.
How AI Algorithms Work
AI algorithms play a crucial role in dynamic pricing strategies by harnessing the power of data and machine learning to make intelligent pricing decisions. These sophisticated algorithms analyze vast amounts of historical and real-time data, such as customer behavior patterns, market trends, competitor prices, and external factors like weather or time of day.
One notable example is web scraping APIs and RPA bots to gather live information about competitor prices on various online sales platforms. By continuously feeding this information into their AI algorithms, companies can ensure they stay competitive while maximizing profits.
Meanwhile, Thompson sampling – a popular method in Bayesian models – allows businesses to balance exploration (testing new price points) with exploitation (leveraging proven price points) based on collected data.
Machine Learning And Data-driven Insights
Machine learning plays a crucial role in the effectiveness of dynamic pricing algorithms by analyzing vast amounts of historical and real-time data to generate accurate insights for optimal pricing decisions.
By continuously processing large datasets, these advanced algorithms become even more refined and adept at predicting patterns and trends in consumer behavior, supply chain dynamics, and market fluctuations.
For instance, let’s consider an e-commerce company that wants to implement a dynamic pricing algorithm. The AI system first gathers essential data points such as competitor prices, seasonal demand changes, customer preferences, purchasing habits, and inventory levels.
Leveraging machine learning technologies like neural networks or Thompson sampling techniques will enable the algorithm to efficiently process this colossal amount of data.
In summary, machine learning solutions significantly enhance data-driven decision-making in dynamic pricing strategies by providing valuable insights from comprehensive historical trends and real-time market conditions analyses.
Different Models Of Dynamic Pricing AI
Dynamic pricing AI includes various models such as Bayesian, Reinforcement Learning, and Decision Tree.
Bayesian Model
The Bayesian model is a dynamic pricing algorithm that uses historical data to determine the optimal price for a product or service. This approach assigns probabilities to pricing options based on previous sales and market trends.
For example, if a certain product sold well at $10 in the past, the Bayesian model would assign a higher probability to that price point than to other potential prices.
This approach has been used successfully in industries like e-commerce, where prices can change frequently due to demand fluctuations and competition. With the help of machine learning algorithms, businesses can continuously gather information and refine their pricing strategies over time.
Reinforcement Learning Model
Another model for implementing dynamic pricing AI is the reinforcement learning model. This model uses artificial intelligence to optimize prices by learning from customer behavior and adjusting prices accordingly.
For example, an e-commerce retailer wants to optimize its pricing strategy using a reinforcement learning model. The algorithm would first set a starting price for each product.
As customers interact with the site, the algorithm will adjust prices based on their purchasing behavior.
Overall, reinforcement learning models offer businesses an effective way to learn from customer data in real time and continually improve their pricing strategies based on insights gained over time.
Decision Tree Model
Decision tree models are dynamic pricing AI that uses a binary tree structure to predict the best price for an item or service. These models break down possible actions and outcomes into branches, allowing businesses to make informed decisions based on data-driven insights.
The advantage of decision tree models is their ability to provide transparent recommendations based on specific business needs, such as revenue maximization, profit maximization, or customer churn minimization.
Modules For Implementing Dynamic Pricing AI
There are several modules for implementing dynamic pricing AI, including the long tail module, elasticity module, key value items (KVI) module, competitive-response module, omnichannel module, time-based pricing module, and conversion rate pricing module.
Long Tail Module
The Long Tail module is one of the Modules for Implementing Dynamic Pricing AI. To determine pricing strategies, it is designed to benefit new or longtail products with little to no historical data.
For instance, businesses that engage in much experimentation and often introduce new products into their mix will find this module particularly useful. Traditional pricing models may not provide accurate results without sufficient sales history for these items.
One way dynamic pricing algorithms use historic sales and price data to inform their decisions about product prices is through modules like the Longtail module.
Elasticity Module
The Elasticity Module is a crucial component of dynamic pricing algorithms that helps businesses calculate the impact of price on demand. By analyzing historical and real-time data, this module considers factors such as seasonality and cannibalization to determine how price changes affect customer demand.
For example, if a retailer notices an increase in sales during holiday seasons or weekends, they can adjust their price accordingly to maximize revenue while maintaining customer loyalty.
One leading Asian e-commerce player saw significant results from implementing the Elasticity Module, resulting in a 10% increase in gross margin and a 3% growth in GMV.
Key Value Items (KVI) Module
The Key Value Items (KVI) module is crucial to implementing dynamic pricing AI. It uses statistical scoring to determine the importance of each item to consumer price perception, helping businesses align their pricing strategies with customer needs and preferences.
This module has been proven effective in improving customer price perception for leading European nonfood retailers. By choosing prices that maximize revenue and profit, minimize customer churn, and align with business objectives, businesses can achieve better sales results using KVI’s data-driven decision-making capabilities.
The KVI module acts as a powerful tool for adjusting prices dynamically based on real-time market trends.
With the power of dynamic pricing algorithms combined with the Key Value Items (KVI) module, businesses are able to create personalized experiences for customers while maximizing profits using real-time data about competitors’ prices collected by web scrapers, APIs, or RPA bots providing valuable information from different sources at once.
Bayesian models and reinforcement learning models are popular dynamic pricing algorithm types used by businesses due to their ability to analyze customer behavior patterns efficiently, resulting in effective personalized prices, which reduces customer churn significantly, ensuring continued patronage from loyal consumers over time.
Competitive-response Module
The competitive-response module is a critical part of dynamic pricing AI used to optimize pricing strategies. By leveraging granular pricing data from competitors, the module analyzes the impact of those prices on a company’s customers and reacts to competitor prices in real time.
For instance, in the e-commerce industry, the competitive-response module monitors competitors’ sites for price changes and adjusts its own site accordingly. If another retailer offers a product at a lower price than you, this algorithm can respond quickly by reducing your price to keep up with the competition while maintaining profit margins.
The goal is to compete and maximize revenue while remaining cost-effective based on historical sales and current market demand analysis.
Omnichannel Module
The Omnichannel module is crucial to implementing Dynamic Pricing AI for businesses. This module considers the customers’ shopping behavior across different channels, such as online and offline stores, to set optimized prices.
It takes into account factors like product availability, promotions, and customer preferences to determine prices that are both competitive and profitable.
This module’s use allows businesses to provide seamless purchasing experiences across all channels while also ensuring competitive pricing strategies across these platforms.
By accurately predicting consumer behavior and adjusting prices based on real-time data extracted by tools like Bright Data’s Web Scraper or RPA bots, dynamic pricing algorithms with an Omnichannel module can help increase revenue while maintaining brand loyalty.
Time-based Pricing Module
The time-based pricing module is a powerful tool designed to help retailers adjust their prices based on time-sensitive factors. With the integration of AI-powered tools, this module can efficiently boost revenue and gross profit for businesses.
For example, if an e-commerce store experiences an unexpected surge in demand during peak holiday shopping seasons like Black Friday or Cyber Monday, they can use this technology to raise prices temporarily and maximize profits.
Additionally, the module helps retailers monitor and respond to competitor behavior by adjusting their prices in real time accordingly.
Conversion Rate Pricing Module
The conversion rate pricing module is a vital component of implementing dynamic pricing AI. This module helps businesses optimize prices at the product level by analyzing customer behavior and purchase patterns.
By understanding how different price points influence customer buying decisions, businesses can adjust their prices to maximize conversions and profits.
One company that uses the conversion rate pricing module is Imprice, a cloud-based dynamic pricing platform that helps retailers optimize their prices using AI. With Imprice’s conversion rate optimization capabilities, businesses can identify which products have low or stagnant sales rates and adjust their prices accordingly.
Advantages Of AI-Optimized Pricing Strategies
AI-optimized pricing strategies maximize revenue and profit, minimize customer churn, compete with competitors’ prices, improve customer experience and loyalty, and align with business objectives.
Maximize Revenue And Profit
AI-optimized dynamic pricing strategies can help businesses to maximize revenue and profit. By using historical and real-time data, AI algorithms analyze market trends, competitor prices, customer behavior patterns, and demand fluctuations to adjust prices accordingly.
For example, Imprice’s AI-powered platform offers modules such as KVI pricing and competitive-response pricing that allow retailers to optimize their prices for individual products based on market conditions.
As a result, businesses can increase their margins without sacrificing sales volume or customer satisfaction.
With AI-driven dynamic pricing strategies at their disposal, businesses can better compete in today’s fast-paced digital marketplace by maximizing revenue and profits while enhancing customer experience through personalized pricing models tailored to each individual consumer.
Minimize Customer Churn
Minimizing customer churn is a crucial aspect of any business, and dynamic pricing AI can help achieve this goal. With personalized prices based on customer behavior and preferences, businesses can keep their customers engaged and prevent them from switching to competitors.
Dynamic pricing also enables real-time adjustments to prices based on market demand, preventing customers from feeling like they are overpaying or missing out on deals elsewhere.
By keeping prices competitive and relevant, businesses can maintain customer trust and loyalty while maximizing revenue and profit.
Compete With Competitor Prices And Attract Their Customers
One of the key advantages of implementing dynamic pricing AI is the ability to compete with competitor prices and attract customers. With real-time data analysis, businesses can adjust their prices to match or even beat those of their competitors, increasing their chances of winning over new customers.
For example, imagine a hotel that uses dynamic pricing AI to adjust its rates based on market demand in real time. The hotel’s algorithm analyzes its competitor’s prices and offers discounted rates that match or beat them during off-peak periods.
As a result, potential guests who were considering other hotels may be swayed by the more attractive rates offered by this hotel, thereby increasing bookings and revenue.
Improve Customer Experience And Maintain Loyalty
One of the most significant advantages of implementing AI-based dynamic pricing strategies is improving customer experience and maintaining loyalty. With personalized pricing, customers can feel that they are being offered fair prices based on their preferences and past behaviors.
For example, let’s say a hotel implements a dynamic pricing strategy that offers lower rates during off-peak seasons while keeping prices high during peak seasons or major events.
By using data-driven insights from machine learning algorithms, businesses can offer targeted promotions and discounts to specific customers, increasing their satisfaction with the brand even further.
Align With Business Objectives
Ensuring that dynamic pricing strategies align with business objectives is crucial for the success of any company. It means taking into account factors such as revenue goals, market share, competitive positioning, and customer satisfaction when optimizing prices with AI algorithms.
One example of successful dynamic pricing implementation is Uber. Their objective was to maintain their position as a leader in ride-sharing services while providing affordable rates for customers and fair compensation for drivers.
To achieve these goals, they utilize real-time data on supply and demand patterns to set surge prices during peak hours automatically.
Industries That Use Dynamic Pricing AI
Industries such as airlines, e-commerce, hospitality, car rental services, and ride-sharing companies like Uber have all implemented dynamic pricing algorithms to optimize their pricing strategy.
Airlines
Airlines were among the first industries to adopt dynamic pricing algorithms, and it’s no surprise that they continue to rely heavily on them today. Dynamic pricing algorithms allow airlines to adjust their fares according to market demand, allowing them to maximize revenue without sacrificing too many empty seats.
For example, airlines may increase prices for flights during peak seasons or when demand is high while lowering prices for less popular routes or off-peak times. Additionally, dynamic pricing allows airlines to adapt quickly to changes in the market and customer behavior, such as sudden shifts in demand due to unexpected events like pandemics or natural disasters.
E-commerce
E-commerce businesses are some of the most prominent users of dynamic pricing AI. With competition growing steeper, these companies need to optimize their pricing strategies continually.
Dynamic pricing algorithms in e-commerce use machine learning and extensive data analysis to predict market trends, analyze customer behavior, and evaluate competitor prices to provide personalized pricing decisions that help maximize revenue and minimize customer churn.
Platforms like Imprice allow businesses to optimize prices at the assortment level and offer a library of pricing strategies for various drivers like sales objectives, competitive response, or omnichannel strategy.
Hospitality
Dynamic pricing is widely used in the hospitality industry to optimize pricing strategies and increase profits. By leveraging data about market trends, customer behavior, and production costs, AI-driven algorithms can suggest price adjustments that align with corporate goals and improve the overall customer experience.
For example, time-based pricing is commonly used in hospitality to increase profitability based on seasonality. Hotels may charge higher rates during peak vacation periods while offering special promotions during slower business months.
Car Rental
The car rental industry has utilized dynamic pricing algorithms to optimize its pricing strategies. By analyzing historical data, real-time competitor prices, and customer behavior, car rental companies can adjust their prices in real-time to capture maximum revenues and profits.
For instance, a car rental company may use the elasticity module to adapt its rates according to demand fluctuations or use the competitive-response module to match its prices.
Dynamic pricing allows businesses in the car rental industry to offer competitive rates while maintaining profitability by tailoring their prices based on various factors such as seasonality, location-based demand, and inventory availability.
How To Choose The Most Suitable Dynamic Pricing AI For Your Business
To choose the most suitable dynamic pricing AI for your business, consider the following:
- Business objectives: Ensure your chosen dynamic pricing algorithm aligns with your business goals of revenue or profit maximization, customer retention, or market share growth.
- Customer behavior: Choose an algorithm that tracks customer browsing and purchase history to offer personalized prices and deals.
- Competitor prices: Look for a tool that scrapes real-time data from competitors’ websites and adjusts your prices accordingly.
- Data-driven decision-making: Ensure that the algorithm uses machine learning to analyze historical data and market trends to optimize prices instead of relying on pre-set rules.
- Pricing modules: Choose a flexible pricing module that can tailor prices for different product categories, sales channels, and time periods.
- Implementation cost: Consider whether you need a cloud-based or on-premises solution based on the size of your business and IT infrastructure.
- Scalability: Select an algorithm that can scale up or down as needed without compromising performance.
By considering these factors, you can effectively select a dynamic pricing AI solution that fits best with your business needs.
Conclusion
Dynamic pricing AI is a game-changer for businesses looking to optimize their pricing strategies. By leveraging modern algorithms, companies can harness the power of real-time data and machine learning capabilities to optimize dynamic pricing.
These cutting-edge technologies help maximize revenue and profit, minimize customer churn, compete with competitors’ prices and attract customers, improve customer experience and maintain loyalty, and align with business objectives.
With dynamic pricing constantly evolving, businesses need to choose the right algorithm for their needs.
FAQ
What is dynamic pricing in artificial intelligence? How do AI algorithms work to optimize pricing strategies? Which industries use dynamic pricing, and how can businesses choose the most suitable one? These are some of the frequently asked questions about the power of dynamic pricing AI.
What Is Dynamic Pricing In Artificial Intelligence?
Dynamic pricing in artificial intelligence is a data-driven pricing approach involving automated tools to optimize retail prices in real-time. This involves using algorithms and machine learning models that take into account factors such as market trends, production costs, customer demand, and competitor analysis to determine the best price for each product or service.
Dynamic pricing can be used in various industries, such as hospitality, airlines, e-commerce, and car rental. For example, airlines use dynamic pricing to maximize revenue by adjusting ticket prices based on factors like flight time and seat availability.
What Are Examples Of Dynamic Pricing?
Dynamic pricing is a strategy that has been adopted by several industries to optimize their revenue and profit. One of the most common examples of dynamic pricing is in the airline industry, where ticket prices fluctuate based on demand, season, and other factors.
For instance, if you book a flight during peak travel seasons such as Christmas or summer holidays, the ticket price will be much higher than booking it during off-season periods.
Another example of dynamic pricing is used in e-commerce stores like Amazon. The platform uses real-time data algorithms that analyze customer behavior and adjust product prices to match competitors’ prices while offering customers a better deal.
What Are Dynamic Pricing Algorithms?
Dynamic pricing algorithms are sophisticated tools that use historical and real-time data, machine learning, and AI to optimize business pricing strategies. These algorithms help companies set optimal prices based on factors such as demand, competition, customer behavior and preferences, seasonality, and supply chain constraints.
For instance, airlines use dynamic pricing algorithms to adjust ticket prices based on variables like route popularity or time of day. E-commerce websites use these tools to personalize prices dynamically based on user browsing history and purchase patterns.
What Is Dynamic Pricing Technology?
Dynamic pricing technology is a strategy that leverages AI and machine learning capabilities to adjust prices in real time based on market demand, customer behavior, and competitor prices.
This technology inputs data about production costs, historical sales trends, seasonal patterns, and inventory levels to output an optimal price for a particular product or service.
For example, airlines use dynamic pricing algorithms to adjust ticket prices based on factors like weather conditions, flight duration, seat availability, and time of purchase.
Retailers can also leverage this technology with modules such as the Long Tail module or the Competitive Response module offered by Imprice’s cloud-based dynamic pricing platform to help them optimize their own prices while staying competitive with other brands in their industry.
What Is an AI-Based Pricing Strategy?
AI-based pricing strategy is a dynamic pricing approach that uses machine learning and artificial intelligence (AI) algorithms to optimize prices. This method analyzes data in real-time, such as customer behavior, competitor prices, market demand, and historical sales data, to determine the best price for a product or service.
AI-based pricing strategies aim to maximize revenue while maintaining customer satisfaction by offering personalized rates and discounts. Examples of industries that use AI-based pricing include airlines, e-commerce platforms like Amazon, hospitality providers such as hotels, and car rental services.
Is Dynamic Pricing A Good Strategy?
Dynamic pricing is a proven strategy that can help companies optimize their prices and increase profitability. By using AI algorithms to analyze market trends, historical data, and real-time data, dynamic pricing can provide personalized prices for customers and anticipate competitor prices.
Although there have been concerns about transparency and job displacement with AI in pricing optimization, dynamic pricing remains an effective approach for businesses seeking to maximize revenue while minimizing costs.
How does dynamic pricing AI work?
Dynamic pricing AI works by collecting data from various sources, such as historical sales patterns, competitor prices, inventory levels, and customer behavior. The algorithm analyzes this information to determine the optimal price for each product or service at any given time. As the data changes over time, the algorithm updates its calculations accordingly to ensure the price remains competitive while maximizing revenue.
Is implementing dynamic pricing AI complicated?
Implementing dynamic pricing AI can be complex, but many software solutions are available that make it easier for businesses of all sizes to adopt this technology without the extensive technical expertise required.
Will using dynamic pricing strategies alienate loyal customers who are used to paying a set price for products or services?
It depends on how you implement your new strategy – if done correctly through targeted messaging & promotions aimed at educating customers about value propositions associated with fluctuating costs, then typically, consumers respond positively when they perceive organizations as taking advantage of them due to unpredictable fluctuations rates purchases otherwise may go overlooked altogether!