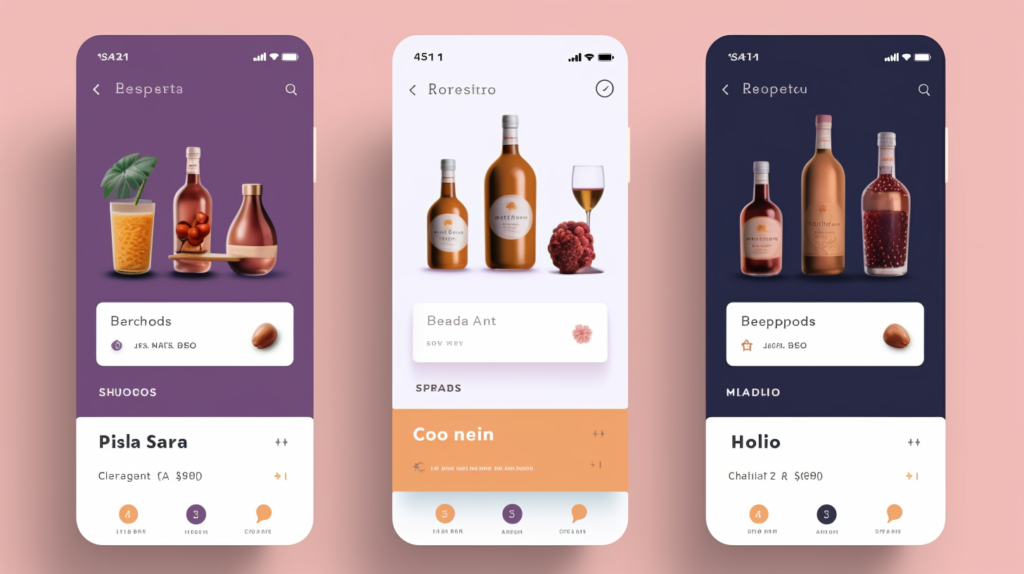
AI product recommendation refers to using advanced artificial intelligence (AI) technology to suggest highly relevant and tailored items to individual customers.
One prominent example of a successful AI-based recommendation system is Amazon’s “Frequently Bought Together” feature. By leveraging machine learning algorithms and analyzing customer behavior, Amazon can identify products often purchased by other users, resulting in highly accurate cross-sell and upsell suggestions for new customers.
These cutting-edge systems go beyond traditional hard-coded rules or basic collaborative filtering methods. Incorporating big data analytics techniques such as natural language processing (NLP), deep learning models, and predictive personalization tools into their frameworks provides more dynamic results that can adapt quickly to changing consumer interests.
Table of Contents
Importance Of AI In Product Recommendations
AI is crucial for product recommendations as it provides personalization and customer engagement, improves conversion rates and customer loyalty, and offers efficient and scalable solutions.
Personalization And Customer Engagement
Personalization is tailoring content, product offerings, and user experiences to individual customer preferences, behaviors, and needs. By leveraging AI-driven product recommendations, businesses can create personalized shopping experiences that are highly engaging for customers.
Customer engagement is crucial in today’s competitive eCommerce landscape because it fosters a strong relationship between the business and its consumers. A simple example comes from Netflix – their use of AI-powered recommendations allows them to provide each viewer with unique content suggestions based on their viewing habits so that users continue watching and interacting with their platform.
Improved Conversion Rates And Customer Loyalty
AI-driven product recommendations have been proven to significantly increase conversion rates and boost customer loyalty. By leveraging the power of machine learning algorithms and analyzing vast amounts of customer data, these recommendation systems can provide personalized suggestions that resonate with each user’s unique preferences and needs.
Improved conversion rates are achieved through making relevant product suggestions and strategically implementing cross-selling and upselling opportunities based on insights gained from customer behavior patterns.
Meanwhile, boosting customer loyalty stems from understanding their preferences over time, enabling businesses to offer products perfectly aligned with their tastes. This increased personalization strengthens the emotional connection between customers and brands, nurturing long-lasting relationships that lead to higher retention rates and recurrent purchases.
Efficient And Scalable Solutions
Efficiency and scalability are critical aspects of AI-powered product recommendation systems, enabling businesses to adapt and grow in response to ever-changing consumer demands and preferences.
With the help of advanced machine learning algorithms, these solutions can quickly process vast amounts of customer data, identifying trends and generating tailored recommendations in real time.
Take Amazon, for example – its cutting-edge recommendation engine analyzes clickstream behavior, transaction history, stock data, social media data, and more to deliver highly personalized suggestions based on individual users’ needs and interests.
This automated approach has proven highly successful; according to McKinsey & Company, research analysis on e-commerce growth rate showed that up to 35% of Amazon’s sales are generated through their personalized recommendations system.
Types Of AI-Powered Recommendation Systems
AI-powered recommendation systems come in various types, including content-based systems that suggest products based on a customer’s past interactions, collaborative filtering systems that rely on similar consumer data to make recommendations, and knowledge-based systems that utilize expert input and domain-specific knowledge to provide suggestions.
Content-Based Systems
Content-based recommendation systems are designed to analyze the attributes of an item and recommend other items with similar properties. These systems make recommendations by identifying patterns in the characteristics of products that a user likes or has interacted with before.
One advantage of content-based systems is that they require very little information and are independent of other users’ data. This makes them easy for unfamiliar users who have just started using e-commerce platforms.
However, these systems can also lead to limiting suggestions because they rely solely on existing data and do not account for changes in user preferences over time.
Collaborative Filtering Systems
Collaborative filtering systems are one of the most popular types of AI-powered recommendation engines. This system works by analyzing the behavior of all users in the past to predict items or ratings that a user may be interested in.
The beauty of this type of system is its ability to provide recommendations for long-tail items without much prior knowledge. Collaborative filtering systems identify item similarities and patterns across millions of customer data points using advanced machine-learning algorithms and data-filtering tools.
Knowledge-Based Systems
Another type of AI-powered recommendation system is the knowledge-based system. Unlike collaborative filtering and content-based systems, knowledge-based systems require information about the subject area to provide recommendations based on complex conditions.
For instance, this system can be applied in medical diagnosis or financial advising, where extensive knowledge is needed to make accurate recommendations. A shoe retailer may use a knowledge-based system to recommend different types of shoes for different foot arch and width measurements.
The downside of these systems is that they require manual input from experts in the field to create rules for decision-making based on the available data.
How AI-Driven Recommendation Systems Work
AI-driven recommendation systems collect and process vast amounts of customer data, analyze and filter it using complex algorithms, and then implement personalized recommendations in real time based on a user’s behavior.
Data Collection And Processing
AI-based recommendation systems rely on data collection and processing to provide personalized product recommendations. Various data collection methods include monitoring user searches and behavior, analyzing social networks, and tracking transactions.
For example, Amazon’s AI recommendation system uses data from its millions of Prime subscribers to track shopping trends. The company analyzes customer behavior, such as what they add to their cart but don’t buy or which products they frequently return, to suggest better items for users through a customized algorithm.
The collected data can be analyzed with machine learning algorithms that use deep-learning models to find patterns in the customer’s activity history, such as transactional history, stock date reviews, etc., allowing businesses to provide personalized recommendations at scale.
Analyzing And Filtering Data
AI-driven product recommendation systems rely heavily on data collection and analysis to make accurate and relevant user suggestions. After the initial data is collected, it goes through a process of filtering out any unnecessary or irrelevant information.
This is achieved using clustering, classification, and association rule mining techniques.
This allows for real-time micro-segmentation where customers are grouped based on their preferences and past activities so that they can be presented with personalized product recommendations.
Implementing Recommendations
After analyzing and filtering data, the next step is to implement recommendations. AI-driven recommendation engines can provide personalized cross-channel experiences such as dynamic ad and email marketing, online store content, and growth marketing strategies.
Another way recommendation engines can improve sales is by offering frequently bought together products or upsell suggestions at checkout. This method has proven effective for retailers using Shopify and Magento platforms, increasing conversion rates by up to 20%.
Real-time micro-segmentation allows marketers to divide their customers into small groups based on demographics, behavior, preferences, transactions, and clickstream activity provided through predictive analytics technology or stock data gathered over time.
Benefits Of Implementing AI-Based Recommendation Systems
AI-powered recommendation systems provide enhanced customer experience, increased sales and revenue, better inventory management, and effective marketing strategies.
Enhanced Customer Experience
One of the main benefits of implementing AI-based recommendation systems is enhanced customer experience. By analyzing customer behavior, preferences, and past purchases, these systems can suggest highly personalized products more likely to interest and delight them.
For example, Amazon Prime uses an advanced recommendation engine that uses machine learning algorithms to analyze user data such as clickstream behavior, transactions, stock data, social media data, and sales history.
This helps Amazon personalize its product recommendations for each individual based on their previous interactions with the site. As a result, customers are more likely to make repeat purchases and stay loyal to the brand.
Increased Sales And Revenue
AI-powered product recommendations have proven to be a game-changer for businesses looking to increase their sales and revenue. By offering personalized product recommendations, e-commerce brands can provide customers with relevant products they may not have discovered otherwise, leading to increased conversions and higher order values.
In fact, according to McKinsey & Company, companies that implement AI-powered recommendation engines can see up to a 30% increase in customer lifetime value and as much as a 20% boost in total sales compared to those without such systems.
Amazon is an excellent example; it attributes up to 35% of its revenue from cross-selling through its “Customers who bought this item also bought” feature.
Better Inventory Management
Implementing AI-powered product recommendation systems can greatly improve inventory management for businesses. By analyzing customer data and behavior, these systems can recommend the most relevant items to customers, making it easier to find products they want.
This increases the chances of a sale and reduces the likelihood of returns due to incorrect recommendations.
Retailers invested nearly $2.5 billion in product recommendation systems in 2019 alone, with the market projected to grow by over 34% from 2020-2025 based on an increased need for customer retention and return on investment (ROI).
Effective Marketing Strategies
One of the significant advantages of AI-based recommendation systems is that they can improve marketing strategies. With the ability to analyze vast amounts of data, businesses can gain insights into customer behavior and preferences, which can be used to create more effective targeted marketing campaigns.
Additionally, personalized cross-channel experiences enabled by AI recommendations have been shown to drive higher conversion rates and increase average order value. By providing relevant product recommendations through dynamic ads, email marketing campaigns, and online store content based on real-time micro-segmentation using machine learning algorithms, brands can better engage customers and boost sales while improving their bottom lines.
Challenges And Considerations In Adopting AI For Product Recommendations
One of the biggest challenges in adopting AI for product recommendations is ensuring data privacy and security while integrating existing systems and evaluating machine learning performance.
Data Privacy And Security
Data privacy and security are major concerns regarding AI-based product recommendations. Companies need access to customer data for their recommendation systems to work effectively, but they are also responsible for protecting that data from cyber attacks and breaches.
Additionally, companies must be careful not to make wrong recommendations that could damage or violate their customers’ trust. The potential consequences of poor recommendations range from minor inconveniences, such as showing ads for items the customer has already purchased, to serious breaches of privacy or safety.
Integration With Existing Systems
Integrating AI-powered product recommendations into existing systems can be a complex process that requires careful consideration. Determining how the recommendation engine will fit within the current infrastructure and ensuring seamless integration with other software or platforms is crucial to avoid disrupting business operations.
Companies may need to invest in new technology or upgrade current systems to support the implementation of recommendation engines.
According to research by IDC, retailers invested nearly $2.5 billion in product recommendation systems in 2019. Despite the potential for enhanced customer experience and increased sales, there are also challenges associated with adopting AI-based product recommendations.
One such challenge is ensuring data privacy and security while collecting user information necessary for effective personalization strategies. Brands also need extensive customer data, including insights from reviews and interactions with customer service representatives, to make accurate recommendations through machine learning algorithms.
Evaluating Machine Learning Performance
One of the challenges in adopting AI for product recommendations is evaluating machine learning performance. It’s important to assess how well the recommendation engine works and whether it’s improving over time or not.
Companies need to establish metrics to measure, such as click-through rates, conversion rates, and revenue generated by personalized recommendations.
Moreover, brands can use scenario testing to evaluate how a recommendation engine performs in different scenarios and ensure that all possible use cases are covered before deploying it.
Considering data on transactions, clickstream behavior, stock data, social media data, and retailers’ commercial priorities while developing machine learning models for personalization can also enhance its efficacy.
Conclusion
In conclusion, AI-powered product recommendations have become essential for eCommerce retailers to enhance their customer experience and drive sales revenue. With the rise of big data and advancements in AI technology, brands can now efficiently provide personalized recommendations by analyzing a vast range of customer data.
They can use algorithms like collaborative filtering or content-based systems to make targeted product suggestions to consumers based on their behavior and preferences.
While implementing an AI recommendation system is not without challenges, such as privacy concerns and machine learning performance evaluations, its benefits are clear: increased customer engagement, loyalty, retention rates, and ultimately better ROI.
FAQ
What Is A Product Recommendation System Using AI?
A product recommendation system using AI is a technology that utilizes machine learning algorithms to provide personalized recommendations to customers based on their preferences, shopping history, and behavior.
– They use various data sources like clickstream behavior, transactions, and social media data to recommend products.
– They can significantly influence online decision-making by displaying alternatives similar to the viewed product.
– They help businesses increase revenue, number of pages viewed, repeat purchases, and average order value.
– Good recommendation engines use real-time micro-segmentation techniques to develop the best product recommendation set for customers.
What Is An Example Of A Recommendation System In AI?
Netflix and Amazon are two popular examples of companies that use AI-powered recommendation systems. Netflix’s system uses machine learning algorithms to analyze a user’s viewing history, ratings, and other data to recommend similar movies or TV shows.
Amazon’s recommendation engine analyzes data from purchases, customer behavior, and product reviews to provide personalized recommendations for items customers might be interested in purchasing
Which Technique Is Used For Product Recommendation?
– Content-Based Systems: These systems recommend products based on analyzing the attributes and features of an item and comparing them to a customer’s previous interactions with similar items.
– Collaborative Filtering Systems: This technique relies on past behavior, such as purchase history, search queries, and browsing activity, to suggest products that customers are likely to buy.
– Knowledge-Based Systems: These systems use customer preferences and additional knowledge about the recommended product or service to generate personalized suggestions based on context.
Overall, the type of recommendation system used depends largely on factors such as diversity, privacy, serendipity, and user demographics, among others.
How does AI technology work to provide product recommendations?
AI algorithms analyze user data, including their search history, demographics, and purchase behavior, to identify patterns and present personalized recommendations based on the individual’s preferences.
Will my privacy be compromised by an AI-based recommendation engine?
Generally speaking, no – information collected is usually opt-in only & governed by strict policies protecting individuals’ safety online. Still, it’s always important to read details when opting into any app or service gathering data so you understand what will be shared/used in order make informed choices as possible regarding one’s own personal information management practices!
Can I easily integrate an AI-based product recommender with my e-commerce website or mobile application?
Yes – many popular e-commerce platforms such as WooCommerce and Magento offer easy-to-use plugins that allow seamless integration of these tools within your existing website structure without requiring major setup changes or technical expertise! It is best practice to consult with an experienced development team early on during project planning stages to ensure implementation meets business needs while complying with industry standards around how consumer data should be handled/stored/transferred safely throughout the process.