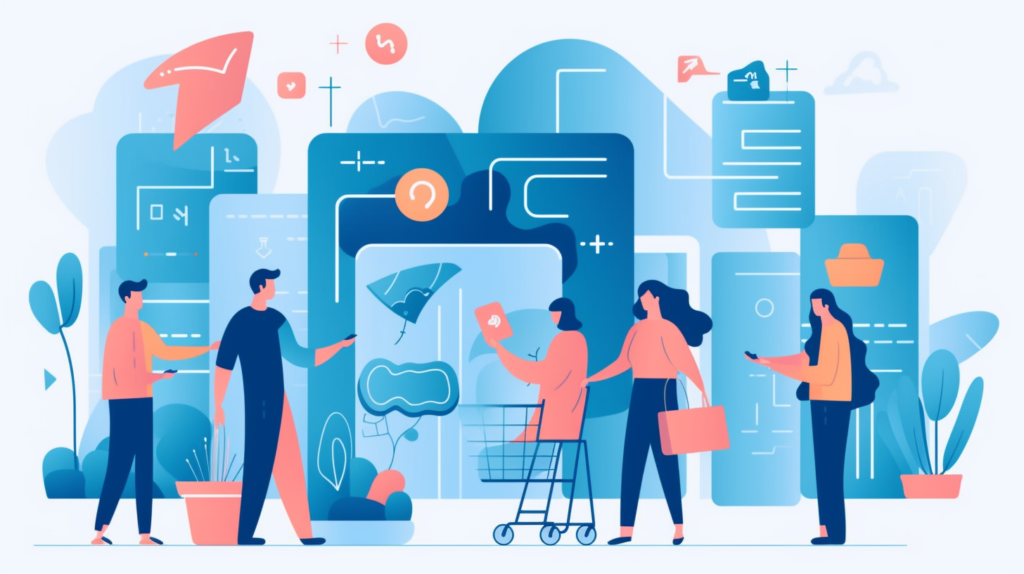
Sentiment analysis, often called opinion mining or emotion detection, is a powerful technique used to identify and categorize subjective information in textual data. For example, consider an eCommerce business that receives thousands of customer reviews daily across multiple platforms, such as social media channels or review websites. Manually analyzing all these comments would be time-consuming and subject to human bias. AI-powered sentiment analysis lets businesses quickly determine whether customers have positive, negative, or neutral feelings about their products or services.
Table of Contents
The Role Of AI In Sentiment Analysis
The role of AI in sentiment analysis has revolutionized how businesses understand and respond to their customers’ emotions, opinions, and overall satisfaction.
A key advantage of using AI in sentiment analysis is its ability to recognize subtle nuances in sentiment- be it sarcasm or mixed feelings – that are often missed by traditional analysis methods.
For example, Coca-Cola utilized IBM Watson’s sentiment analysis capabilities to monitor consumer reactions during a marketing campaign. Their system could accurately distinguish between positive and negative feedback within seconds, making real-time response possible.
Benefits Of AI-Driven Sentiment Analysis In ECommerce
AI-driven sentiment analysis in eCommerce provides various benefits to businesses, including improved customer service through real-time analysis of customer feedback, customized marketing strategies and campaigns based on identified trends and sentiments, enhanced brand reputation monitoring and management through social media insights, as well as better product and service improvements by understanding customer satisfaction levels.
Improved Customer Service
AI-powered sentiment analysis is a game-changer for improving customer service in the eCommerce industry. By quickly and accurately processing vast amounts of unstructured data, such as online reviews, social media comments, and customer support tickets, businesses can gain valuable insights into their customers’ experiences and preferences.
For example, an AI-driven customer sentiment analysis tool could detect a surge in negative feedback regarding slow shipping times or damaged products. Armed with this information, the company can swiftly address these concerns by improving its logistics processes and quality control.
Moreover, conversational guidance platforms help customer service agents respond more effectively to user inquiries using real-time emotion recognition based on text analytics.
Customized Marketing Strategies And Campaigns
Leveraging AI-driven sentiment analysis in eCommerce enables businesses to develop highly customized marketing strategies and campaigns. By analyzing customer feedback, brands can identify trends, preferences, and emotions that help them tailor their advertising efforts to resonate better with their target audiences.
For instance, an online retail company may discover a product feature that elicits positive reactions from customers.
Moreover, AI-based sentiment analysis helps companies monitor social media platforms for real-time responses to new promotional initiatives. They can gather valuable data on consumers’ feelings about specific campaigns or messaging strategies and adjust accordingly.
Enhanced Brand Reputation Monitoring And Management
Using AI-powered sentiment analysis in eCommerce allows businesses to monitor and manage their brand reputation effectively. By analyzing customer feedback and social media mentions, companies can determine how customers perceive their brand and make necessary modifications to improve it.
AI-driven sentiment analysis tools help businesses keep track of online chatter around their products or services, detect negative comments or reviews, appreciate positive ones quickly, and take timely action wherever required.
For example, T-Mobile used sentiment analysis techniques to monitor Twitter conversations about its service during a nationwide network outage. The company’s team responded proactively by providing real-time status updates and addressing customer concerns over social media channels actively.
Better Product And Service Improvements
AI-driven sentiment analysis helps eCommerce businesses improve their products and services by providing valuable customer feedback insights. By analyzing customer sentiment, companies can identify areas for improvement in their offerings, such as feature requests or complaints about product defects.
For example, Coca-Cola leveraged AI-powered sentiment analysis to analyze social media conversations on Twitter surrounding the launch of its new product line. The analyzed data helped the company identify customers’ likes and dislikes about the new soda flavors prompting them to modify certain ingredients based on customers’ preferences before mass production.
Types Of Sentiment Analysis
Learn more about the different types of sentiment analysis, including fine-grained sentiment analysis, emotion detection, aspect-based sentiment analysis, and intent analysis.
Fine-Grained Sentiment Analysis
Fine-grained sentiment analysis is a popular type that categorizes the buyer’s feelings as neutral, positive, or negative. This technique analyzes sentences or phrases and assigns weighted scores to each word.
These scores help measure how positive, negative, or neutral the text is overall.
A good example where fine-grained sentiment analysis can be applied effectively is in an e-commerce business looking to analyze customer feedback, such as product reviews and ratings.
Using this approach enables retailers to extract more detailed information about customers’ experiences than simple polarity-based approaches that only classify feedback as positive or negative.
Emotion Detection
Emotion detection is a type of sentiment analysis that identifies the emotions expressed in text. This can include identifying emotions such as anger, sadness, joy, frustration, and satisfaction in customer feedback.
By pinpointing the specific emotions behind customer feedback, businesses can gain deeper insights into their customers’ experiences and identify areas for improvement. For example, if a customer expresses frustration with a product or service, emotion detection can help businesses understand their frustration and develop targeted solutions to address their concerns.
One real-life use case of emotion detection is Netflix’s recommendation system, which uses natural language processing (NLP) techniques to analyze viewers’ comments on social media platforms like Twitter.
The tool detects viewers’ emotional expressions towards certain movies or TV series, which helps Netflix more effectively personalize its recommendations based on individual viewer preferences.
Aspect-Based Sentiment Analysis
Aspect-Based Sentiment Analysis is a technique e-commerce businesses use to learn more about specific aspects or features of their products and how customers feel about them.
This method allows companies to go beyond general sentiment analysis and delve deeper into what is driving customer satisfaction or dissatisfaction. For example, if a customer leaves a negative review for an online retailer’s clothing item, aspect-based sentiment analysis can help identify whether the issue was with the fabric quality, fit, or design.
Aspect-Based Sentiment Analysis uses machine learning algorithms that learn from feedback and new data over time. This technique has become increasingly popular in the e-commerce industry due to its ability to provide valuable insights quickly on what aspects of their products need improvement.
Intent Analysis
The intent analysis is a specific type of sentiment analysis that focuses on understanding the intent or purpose behind text data. Instead of simply analyzing whether the sentiment expressed in the text is positive, negative, or neutral, the intent analysis aims to determine what action the writer behind the text intends to take.
This can be useful for businesses looking to understand customer behavior and tailor their marketing campaigns accordingly. For example, if a customer leaves a review expressing frustration with slow shipping times, an intent analysis could help determine whether they will switch to a competitor or continue shopping with the company if delivery times improve.
Challenges And Limitations Of AI In Sentiment Analysis
AI-powered sentiment analysis faces challenges and limitations, such as the need for large data, struggles with complex language, and ambiguity in determining sentiment.
Need For Large Amounts Of Data
To train AI-powered sentiment analysis, large amounts of data are needed to optimize and fine-tune the algorithm’s accuracy over time. This means that businesses must have access to vast quantities of customer feedback, online reviews, social media comments, and other unstructured information sources.
For example, a company like Amazon can generate millions of monthly product reviews from its customers worldwide.
Without enough data points for AI-driven sentiment analysis models, the algorithm’s performance may suffer in precision and recall rates. Additionally, smaller datasets could lead to biases or oversimplification in opinion mining tasks when training with pre-trained models or developing custom scoring systems.
Struggles With Complex Language
Despite AI’s advanced capabilities in sentiment analysis, struggles with complex language pose a challenge. Languages such as English can be challenging to analyze due to nuances, sarcasm, and cultural references that may affect the meaning of a text.
To address these challenges in e-commerce customer sentiment analysis using AI technology, companies must employ several strategies, such as machine learning algorithms capable of detecting subtle differences in phrasing or grammatical structure.
They must also consider training their models and developing standard guidelines for analyzing texts across various languages.
Common Tools And Platforms For AI-Powered Sentiment Analysis
There are several tools and platforms available for businesses to conduct AI-powered sentiment analysis, including:
- Google Cloud Natural Language: This platform uses machine learning algorithms to analyze text data from various sources, including social media and customer feedback forms.
- IBM Watson: With advanced natural language processing (NLP) capabilities, IBM Watson is a popular choice for businesses looking to perform sentiment analysis on large volumes of data.
- Amazon Comprehend: This cloud-based service by Amazon Web Services (AWS) offers real-time sentiment analysis and can be integrated with other AWS products like Amazon S3.
- MonkeyLearn: This tool specializes in text analysis and offers pre-trained models for sentiment analysis that can be customized according to specific business needs.
- Microsoft Azure Text Analytics API: Compatible with multiple languages, this API provides a range of NLP services, including sentiment analysis and key phrase extraction.
- Lexalytics: Offering cloud-based and on-premises solutions, Lexalytics has been recognized as a leader in the sentiment analysis market and provides granular insights into customer opinions.
These platforms offer a range of features like real-time analysis, contextual analysis, aspect-based approach, and explainable AI that help provide valuable insights and trends to improve products, services, marketing strategies, and overall operational efficiency.
Case Studies: Successful AI-Powered Sentiment Analysis In ECommerce
Many businesses have successfully implemented AI-powered sentiment analysis to improve their eCommerce operations. Sephora, a cosmetics retailer, used AI-based sentiment analysis to collect and analyze customer feedback from various channels such as social media, email, and online reviews.
The data collected helped the brand identify common customer issues and develop targeted solutions to address them promptly.
Another successful case study is Coca-Cola’s “Share a Coke” campaign, which encouraged customers to personalize the cans with their names or nicknames. Using machine learning algorithms, Coca-Cola analyzed the social media conversations around this campaign through hashtags like #ShareaCoke or #EnjoytheFeeling.
AI-powered sentiment analysis has enabled Airbnb, a popular home-sharing platform, to accurately monitor guest satisfaction levels in real time.
These case studies demonstrate how businesses can use AI-powered sentiment analysis for more effective product improvement strategies while enhancing operational efficiency via better customer service management systems.
Ethical Considerations In Using AI For Sentiment Analysis
As with any use of artificial intelligence, it is crucial to consider the ethical implications of using AI for sentiment analysis. One major concern is avoiding bias in the data collected and analyzed.
This means ensuring that data sets represent all customers, regardless of race, gender, or other factors.
Another important consideration is privacy. Businesses must ensure that customer feedback and personal information are kept secure and not shared without consent.
Human oversight is crucial when using AI for sentiment analysis to ensure accuracy and appropriate results.
Ultimately, using AI for sentiment analysis should be approached with caution and care to avoid unintended consequences and uphold ethical standards while benefiting from improved customer service and understanding customer feedback.
Future Developments And Trends In AI For Customer Sentiment Analysis
The future of AI for customer sentiment analysis looks promising, as new developments and trends are constantly emerging. Natural language processing (NLP) is becoming more sophisticated, allowing for better contextual analysis and the ability to recognize emotions in text.
Moreover, explainable AI is gaining traction as companies seek greater transparency and accountability in using AI for sentiment analysis.
Lastly, no-code sentiment analysis tools are beginning to emerge, allowing businesses without extensive technical expertise to conduct effective sentiment analyses using pre-built models and custom dashboards.
These developments offer exciting opportunities for businesses looking to improve customer experience through advanced AI-powered sentiment analysis techniques.
Conclusion
In conclusion, AI-powered sentiment analysis is a game-changer for the eCommerce industry, providing businesses with valuable insights into customer feedback and improving operations.
With the ability to automatically analyze large volumes of text data in real-time, AI-driven sentiment analysis tools allow companies to tailor their marketing strategies better, enhance brand reputation monitoring and management, and improve customer service.
Although challenges and limitations remain, the future looks bright for AI-driven sentiment analysis in eCommerce.
FAQ
Which Application Of AI Is Used For Customer Sentiment Analysis?
AI-powered sentiment analysis is the primary application used for customer feedback analysis. It involves machine learning algorithms that automatically analyze large volumes of text data and determine the sentiment or overall emotion expressed in the text.
Sentiment analysis provides a richer, more contextual view of content beyond categorizing negative or positive sentiment to identify exact feelings such as sad, happy, excitement, scared, etc. E-commerce analytics is collecting and analyzing data from multiple areas of an online business, with Sentiment Analysis being a key component.
Successful case studies include brands like Airbnb, who have used this technology to improve their products/services.
How Is Sentiment Analysis Used In E-commerce?
Sentiment analysis plays a crucial role in e-commerce by analyzing customer feedback, such as online reviews and comments, to determine overall sentiment towards products or services.
This information helps businesses identify areas for improvement, track brand reputation in real time, and develop effective marketing campaigns that resonate with their target audience.
For instance, Ford uses sentiment analysis to monitor social media conversations about their vehicles and quickly responds to customers’ concerns or questions. Similarly, Coca-Cola used sentiment analysis during the 2014 World Cup to track public opinion about the event on social media channels.
Which Algorithm Is Best For Sentiment Analysis?
Choosing the right algorithm for sentiment analysis largely depends on the specific needs of a business. Rule-based approaches are best suited for simple and straightforward applications, whereas machine learning algorithms can handle more complex cases such as sarcasm and typos.
Hybrid approaches that combine both rule-based and machine-learning methods offer a balance between accuracy and speed. Lexicon-based approaches use pre-existing dictionaries to categorize words based on their sentiment, making them suitable for industries with limited or specialized vocabulary, like banking and healthcare.
When choosing the right algorithm for your business needs, it’s important to consider factors like scalability, data requirements, and training dataset preparation.
Does Facebook Use Sentiment Analysis?
Facebook is one of the companies that uses sentiment analysis to analyze large volumes of data and determine the overall emotion expressed in the text. With over 2.8 billion monthly active users, Facebook collects vast customer feedback, including comments, posts, and messages.
One significant benefit of using AI-powered sentiment analysis for Facebook is real-time brand reputation monitoring.
How Can Sentiment Analysis Be Used By Retailers And Brands?
Sentiment analysis can be a game-changer for retailers and brands. By analyzing customer feedback, businesses can gain insights into their customers’ opinions of products, services, and overall brand experience.
For example, suppose a business analyzes its online reviews using sentiment analysis tools like Google Cloud Natural Language or Microsoft Azure Text Analytics API. In that case, it may uncover recurring complaints about product quality or shipping times.
Armed with this knowledge, the company can address these issues by improving product manufacturing processes or streamlining logistics operations, ultimately leading to increased sales growth.
What is AI-powered customer sentiment analysis in e-commerce?
AI (Artificial Intelligence) powered customer sentiment analysis involves machine learning algorithms to automatically analyze and understand customers’ emotions, opinions, and attitudes towards products or services they purchase online.
How does AI-powered sentiment analysis work for e-commerce businesses?
By leveraging natural language processing (NLP) technology and machine learning models, AI analyzes customers’ reviews, comments, emails, or feedback to identify positive or negative sentiments about a particular product. It then generates actionable insights that can be used by e-commerce businesses to improve their offerings based on what makes customers happy or dissatisfied.
Can AI-based sentiment analysis help increase sales in eCommerce?
Yes! By analyzing customer feedback in real-time using sophisticated algorithms, eCommerce businesses can quickly spot trends that may indicate areas that need improvement and those that are performing well. This enables them to better optimize their marketing campaigns and improve the overall shopping experience, leading to increased sales and revenue.
Are there any ethical concerns linked with using AI for sentiment analysis?
It’s essential for companies utilizing AI for customer sentiment analysis to be transparent with their consumers regarding how data is being collected – and potentially shared amongst other organizations – so users fully understand how it operates before consenting usage through text analytics tools like Zendesk Explore etc. Additionally – given recent controversies surrounding privacy threats from 3rd parties acquiring personal information without consent- ensuring relevant safety measures around access/ sharing protocols should always be implemented beforehand too!