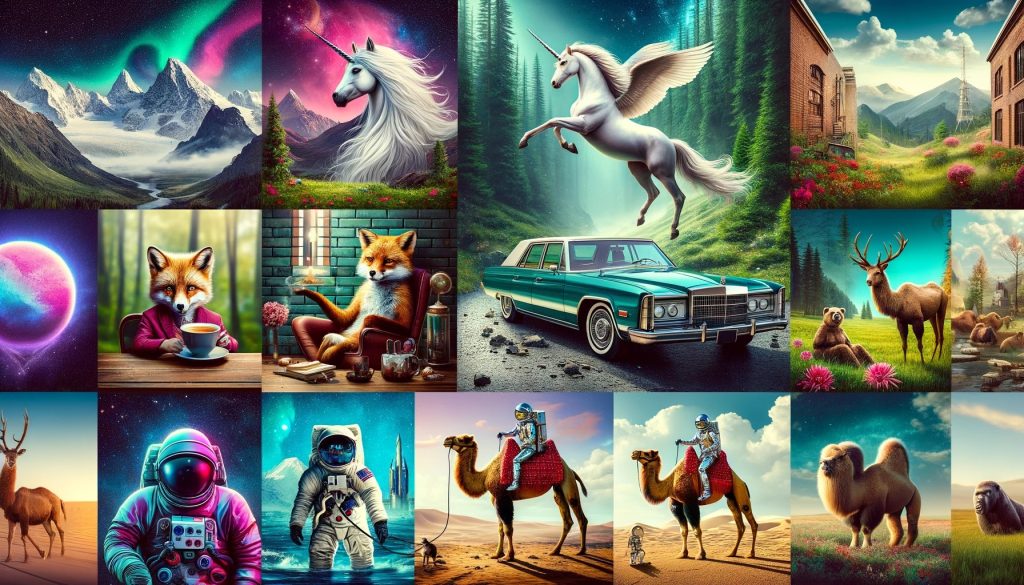
Researchers from the MIT Computer Science and Artificial Intelligence Laboratory (CSAIL) have unveiled a groundbreaking method that significantly accelerates the process of generating high-quality images through artificial intelligence, marking a 30-fold increase in speed. This novel approach, harnessing the Distribution Matching Distillation (DMD) framework, simplifies the traditionally complex and iterative procedure of diffusion models into a singular, efficient step without compromising the quality of the resultant visuals.
Revolutionizing Visual Content Creation
At the heart of this advancement lies a teacher-student model, a strategic simplification where a newly developed computer model learns to replicate the intricate process of more elaborate, original models that produce images. This method, leveraging the principles of both generative adversarial networks (GANs) and diffusion models, paves the way for generating visual content in just one step—contrasting sharply with the hundred steps required by existing diffusion models like Stable Diffusion and DALLE-3.
Key Features of the DMD Framework:
- Efficiency and Speed: DMD accelerates current diffusion models by 30 times, significantly reducing the computational time required to generate images.
- Quality Preservation: Despite the speedup, the quality of the visual content generated remains high, potentially even surpassing that of the original models.
- Simplified Process: By distilling the complex process into a single step, DMD mitigates the instability and mode collapse issues often encountered in GANs.
Implications and Applications
The single-step diffusion model developed through DMD opens up new possibilities for rapid content creation across various fields, including design tools, drug discovery, and 3D modeling. Its capacity for quick and effective visual generation holds promise for significant advancements in how we approach and execute creative and scientific projects.
Distribution Dreams: The DMD Approach
DMD incorporates two main components to achieve its groundbreaking results:
- Regression Loss: This ensures a stable training process by anchoring the mapping for a coarse organization of the image space.
- Distribution Matching Loss: It aligns the probability of generating a particular image with its real-world occurrence frequency, guided by two diffusion models.
This framework has demonstrated its prowess in benchmarks, achieving nearly identical performance to more complex models with a Fréchet inception distance (FID) score of just 0.3. While there remains a slight gap in quality for more challenging text-to-image applications, the achievements of DMD suggest substantial room for future enhancements.
Conclusion and Future Directions
The DMD framework represents a significant leap forward in the field of artificial intelligence and image generation. By combining the versatility and high visual quality of diffusion models with the speed of GANs, this approach sets a new benchmark for real-time visual editing and content creation. As the technology continues to evolve, we can anticipate even greater efficiencies and breakthroughs in high-quality, real-time visual generation.
FAQ
What is the Distribution Matching Distillation (DMD) framework?
DMD is a novel framework that simplifies the image generation process of diffusion models into a single step, accelerating the generation speed by 30 times while maintaining or enhancing image quality.
How does DMD differ from traditional diffusion models?
Unlike traditional multi-step diffusion models, DMD operates in a single step, drastically reducing computational time and complexity. It uses a teacher-student model to replicate the original models’ capabilities more efficiently.
What are the potential applications of the DMD framework?
DMD’s fast and efficient image generation process can benefit various fields, including digital content creation, drug discovery, and 3D modeling, by enabling quicker design iterations and innovations.
How does DMD maintain image quality?
DMD maintains high-quality image generation through a careful distillation process that captures the essence of the original, more complex diffusion models, ensuring that the visual content remains rich and detailed.