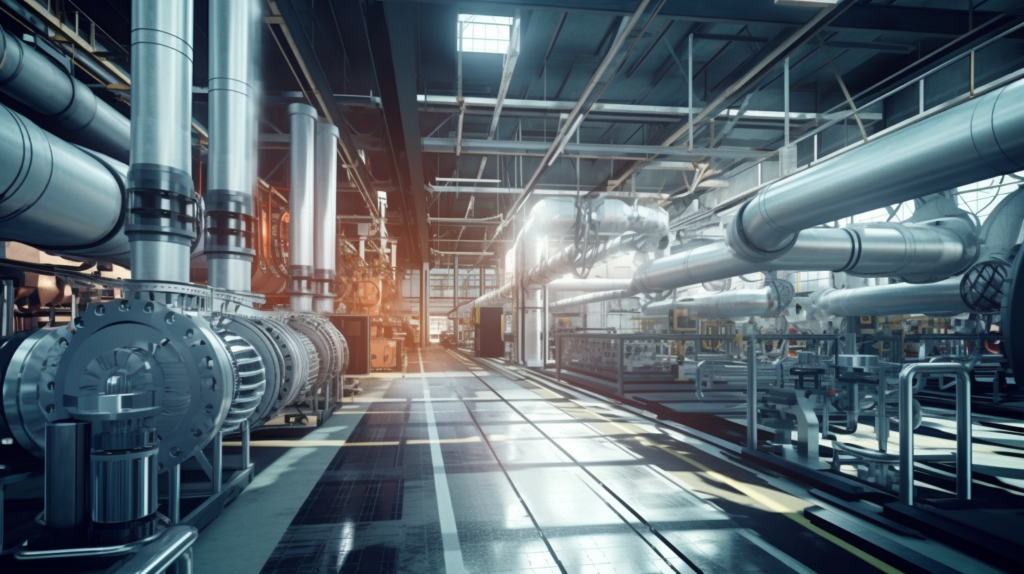
The growing global waste crisis and its impact on our environment have led to a surge in innovation, with artificial intelligence (AI) playing an increasingly crucial role in waste reduction and material efficiency manufacturing. By combining AI technology with robotics, new solutions to efficiently sort plastics, optimize supply chains, and reduce errors in production are emerging. We’ll explore how AI-assisted techniques benefit the environment by minimizing waste and improving the economic performance of businesses.
Table of Contents
Key Takeaways
- AI-powered waste reduction and material efficiency manufacturing can help combat the global waste crisis while improving economic outcomes.
- Machine learning algorithms and robotics are being used to optimize supply chains, reduce wasteful errors, and enhance pollution removal efforts.
- Robotic sorting systems and chemical recycling technologies offer significant potential for reducing plastic waste and promoting sustainability in manufacturing processes.
- Smart solutions for urban waste reduction using AI technology could also be implemented to effectively manage massive amounts of waste generated by cities.
The Growing Problem Of Waste In Manufacturing
The manufacturing industry has been experiencing a significant waste problem, contributing to environmental issues such as pollution and overflowing landfills. With the United States producing the highest waste-to-population ratio at 12% and India and China generating 27% of the world’s waste despite having only 36% of the global population, it is evident that this issue requires immediate attention.
One major challenge manufacturers face is managing their plastic usage and disposal since less than 8.4% of plastics are recycled globally. High levels of poor-quality feedstock and contamination result in discrepancies in recycled materials’ quality, further exacerbating waste management problems within manufacturing sectors.
Due to these challenges, innovative AI and robotics solutions have emerged to effectively reduce landfill deposits while offering considerable cost savings.
These advanced technologies bring forth new opportunities for optimizing material efficiency during production processes while enhancing pollution removal efforts – providing both environmental benefits and financial incentives through improved return on investment (ROI).
AI-Assisted Waste Reduction
AI technology is being used to revolutionize waste reduction strategies in the manufacturing industry, from machine learning sorting systems to robotic recycling technologies.
Machine Learning In Waste Sorting
Machine learning and artificial intelligence are revolutionizing the waste management industry by improving efficiency in sorting processes. As a growing problem worldwide, mismanaged trash can have devastating consequences on our environment.
One example of machine learning’s success in waste sorting can be seen with AMP Robotics’ smart recycling systems. These advanced technologies utilize computer vision, robotics, and optical sorters to accurately identify various types of materials – such as plastics or metals – resulting in a more efficient separating process.
The system rapidly learns from its mistakes while continuously adjusting its recognition software accordingly.
By incorporating AI-powered innovations like machine learning algorithms into waste management practices, both environmental and economic benefits become feasible outcomes.
Reduction in contamination levels leads to higher-quality recovered materials that meet consumer demands without posing health risks associated with improper disposal methods or mixed recyclates usage.
Robotics In Recycling
The integration of robotics in recycling has revolutionized the waste management industry, significantly improving sorting efficiencies and reducing human error. By leveraging advanced artificial intelligence (AI), machine learning algorithms, and computer vision systems, robotic recycling systems can accurately recognize and sort various types of materials, leading to more efficient material recovery at facilities.
Robots operate at speeds roughly twice that of humans while maintaining high precision and quality control levels. This reduces the risk of injury for workers traditionally exposed to hazardous conditions and saves time by speeding up the process.
Innovations in robotic recycling have extended beyond traditional material recovery facilities (MRFs) into other areas, such as ocean plastic cleanup efforts through drones or autonomous vehicles for monitoring landfill sites.
Material Efficiency In Manufacturing
Manufacturing can benefit from material efficiency by optimizing supply chains, reducing wasteful errors, and improving productivity while minimizing environmental impact.
Optimizing Supply Chains
One of the benefits of AI in waste reduction and material efficiency is the optimization of supply chains. Predictive analytics can help manufacturers better understand demand shifts and tailor production accordingly, reducing wasteful overproduction and excess inventory.
This has a significant impact on both environmental sustainability and economic profitability.
Notably, closed-loop supply chain systems that utilize AI technology minimize waste by recycling materials back into production.
Reducing Wasteful Errors
AI-powered technologies are revolutionizing manufacturing by reducing wasteful errors and improving material efficiency. Machine vision systems, for example, can detect quality defects in products and alert operators before they become widespread.
Predictive analytics can also optimize production processes to prevent overproduction or underutilization of materials, reducing waste from the start. Furthermore, automation reduces the risk of human error in sorting materials or performing repetitive tasks that might lead to incorrect outcomes.
Overall, AI technology empowers manufacturers to achieve greater accuracy while using fewer resources, resulting in reduced waste and more sustainable operations.
AI-Powered Innovations In Waste Management
AI-powered innovations in waste management, such as robotic sorting systems and chemical recycling technologies, are revolutionizing the industry by reducing plastic consumption, enhancing pollution removal efforts, and improving return on investment.
Robotic Sorting Systems
Robotic sorting systems have become an increasingly valuable tool in waste management, using artificial intelligence and robotics to sort plastics more efficiently and accurately.
This method reduces the reliance on manual sorters, who are twice as likely to be injured while working.
Facilities that process significant amounts of material justify the cost of implementing robotic sorting systems, with companies such as Glacier, AMP Robotics, CleanRobotics, ZenRobotics, Greyparrot, and Everest Labs having developed AI-powered robotic sorting systems for waste management.
These robotic systems ensure quicker, more precise picking-out of plastics that are then sent into their respective bins.
Overall automation produces a higher quality end product increasing revenue opportunities for facilities; TrashBot is a smart recycling bin developed by CleanRobotics that sorts waste at an accuracy rate of 95 percent using recycling AI technology alongside computer vision technology driven through machine learning algorithms providing efficient processes with precise analytics across every part of the production cycle reducing unnecessary waste resulting in environmental cleanup efforts while minimizing economic impact related costs.
Chemical Recycling Technologies
Chemical recycling is seen as an alternative to traditional recycling methods due to plastic’s destructive nature. It involves breaking down plastic into its individual chemical components, which can then be used to create new materials.
AI-powered innovations are playing a key role in advancing chemical recycling technologies. Machine learning algorithms and machine vision systems are being used to analyze the composition of different types of plastics and identify the most efficient ways to break them down.
Overall, chemical recycling technologies offer great potential for reducing plastic waste and promoting sustainability in manufacturing processes.
Smart Solutions For Urban Waste Reduction
One of the areas where AI-powered waste management shows significant potential is in reducing urban waste. Cities generate vast amounts of waste, and traditional methods often fall short of managing them efficiently.
For example, smart bins equipped with sensors that use machine learning algorithms to separate different types of waste could be placed strategically throughout cities.
Another innovative solution is using computer vision technology to detect contamination in recyclables before it reaches material recovery facilities (MRFs). MRFs sort out recyclable materials from mixed waste but are prone to inefficiencies due to human error or mixups.
Implementing AI-powered solutions for urban waste reduction has many benefits, like improving recycling rates and reducing landfill usage, which prevents greenhouse gas production from decomposing trash.
Environmental And Economic Benefits Of AI-Assisted Waste Reduction
AI-assisted waste reduction can reduce plastic consumption, enhance pollution removal efforts, and improve return on investment for recycling facilities.
Reduced Plastic Consumption
AI technology can assist in reducing plastic consumption by analyzing and simulating different packaging designs to achieve the same strength with less material. By using predictive analytics, companies can better understand demand shifts, avoiding overproduction and reducing plastic waste.
One inspiring example is the End Plastic Waste Initiative launched in 2019 by thirty leading global companies focused on solving plastic waste environmental concerns through partnerships with governments, NGOs, and developers of plastics technologies such as AI and robotics solutions.
Enhanced Pollution Removal Efforts
AI-assisted waste reduction can enhance pollution removal efforts by providing advanced technology to identify and sort different types of materials with high precision. Using computer vision and machine learning algorithms, machines can analyze massive amounts of data that would be impossible for humans to process.
One great example is AMP Robotics’ “Smart Sort” system which uses robotic systems with cameras to recognize individual items on a conveyor belt at lightning speed. The camera delivers an image to the AI-powered program that identifies the object’s material composition instantly.
It then directs robotic armatures precisely position each item into designated bins during recycling processing.
Improved Return On Investment
AI-assisted waste reduction and material efficiency in manufacturing can significantly improve return on investment (ROI). By implementing AI-driven automation, sorting accuracy and efficiency go up significantly, leading to higher revenue opportunities.
Additionally, the reduced labor costs that come with replacing human workers with robots help facilities save money. One example is an MRF that was able to sort 55% more materials while reducing its headcount by half.
An AI investment leads to an ROI in multiple areas for MRFs – from freeing up workers for other roles to preventing safety incidents and improving recycling quality.
Challenges And Limitations Of AI In Waste Management
Implementing AI in waste management is not without its challenges, including technical difficulties and high initial costs. Balancing automation with human labor also poses a challenge.
However, these limitations are outweighed by the numerous benefits that come with AI-assisted waste reduction and material-efficiency manufacturing.
Technical Difficulties
One of the main challenges facing the implementation of AI in waste management is the need for accurate and reliable data. Without a sufficient amount of high-quality input, machine learning algorithms cannot provide useful insights or perform their intended functions effectively.
Furthermore, specialized technical expertise is required to successfully implement AI in waste management. Staff must be trained in correctly operating robotic systems and interpreting results from computer vision systems.
High initial investment costs are also a significant hurdle for many organizations looking to implement this technology.
High Initial Costs
One significant challenge in implementing AI technology for waste management is its high initial costs. The cost of purchasing and installing robotic sorting systems, waste recognition software, and other necessary equipment can be prohibitive for some facilities.
However, while these costs may initially be a barrier to entry, the potential long-term benefits make it a worthwhile investment. For example, using robotics reduces labor costs and efficiency by reducing the reliance on manual sorters.
Additionally, AI data can help MRF owners reduce landfill fees and recoup lost commodity revenue.
Balancing Automation And Human Labor
Incorporating AI in waste management has the potential to improve efficiency and reduce costs, but it’s critical to strike a balance between automation and human labor. While robots can work twice as fast as humans and pick out plastics more precisely, facilities must process significant amounts of material to justify their cost.
Furthermore, human workers play an essential role in interpreting data gathered by AI systems. They provide context that AI algorithms may lack, making it easier to identify the most effective ways of reducing waste.
Balancing automation with human labor is crucial for creating more sustainable manufacturing processes.
Case Studies Of AI In Waste Management
Some successful case studies of AI in waste management include CleanRobotics’ “Trashbot,” which uses computer vision and machine learning to sort recyclables, AMP Robotics’ robotic sorting systems that can quickly and accurately sort materials, Everest Labs’ ultrasonic technology for detecting contaminants in recycling streams, and Greyparrot’s deep learning algorithms for identifying different types of plastic.
Waste Sorting Startups
Waste sorting startups are using AI technology to revolutionize the waste management industry. Companies like AMP Robotics, CleanRobotics, ZenRobotics, Greyparrot, and Everest Labs are implementing machine learning and computer vision systems to sort recyclables more efficiently than ever.
These technologies can quickly distinguish between different materials, speeding up the sorting process and reducing contamination. Additionally, AI enables these startups to recover more valuable recyclables from the waste stream while also improving worker safety by replacing manual sorting processes with robotic solutions.
AI-Based Recycling Programs
AI-based recycling programs are becoming an essential solution to the plastic sorting crisis, with a predicted 12 billion metric tons of plastic in landfills by 2050. Recycling centers are implementing AI technology and robotics to improve waste management efficiency, reduce contamination, and enhance pollution removal efforts.
One such program is the TrashBot developed by CleanRobotics, which utilizes recycling AI, computer vision systems, and machine learning algorithms to sort waste with 95% accuracy while reducing human error.
The AMP Robotics system uses robotic arms equipped with cameras and sensors that can accurately identify plastics at different angles for accurate separation.
Future Predictions For AI In Waste Reduction And Material Efficiency
The future of AI in waste reduction and material efficiency looks promising. With the increasing number of companies adopting AI technologies to manage their waste, there is a growing demand for machine vision systems and predictive analytics that can more accurately predict what types of materials will end up in landfills.
Another development expected in the near future is the rise of closed-loop supply chains. By using advanced sensors and tracking technology, manufacturers can monitor production processes from start to finish, reducing wasteful errors caused by human error or inefficient supply chains.
Finally, smart cities will play a role in driving AI adoption for sustainable waste management practices. Cities around the world are investing heavily in smart infrastructure, such as automated waste collection systems that use computer vision to sort trash on the spot before being transported away for processing.
Overall, we see numerous potential applications for AI technology across all facets of addressing our plastic problem; however, it must be acknowledged that there remain significant challenges ahead – including technical limitations with current sensing technologies – as well as important moral questions surrounding how best this powerful tool should be used within society’s sustainability efforts moving forward.
Conclusion
AI-assisted waste reduction and material efficiency manufacturing is the future of the industry. With growing concerns about plastic waste and pollution, implementing AI technology can help reduce negative environmental impacts while improving economic outcomes.
From machine learning in waste sorting to robotic recycling, there are innovative solutions available for optimizing supply chains, reducing wasteful errors, and improving overall efficiency.
Although challenges and limitations remain with balancing automation and human labor, AI-driven automation offers immense benefits, including enhanced safety measures, improved return on investment, and reduced costs.
FAQ
What is AI-assisted waste reduction and material efficiency manufacturing?
AI-assisted waste reduction and material efficiency manufacturing refers to using artificial intelligence (AI) technologies, such as machine learning algorithms, to optimize production processes to reduce waste and maximize resource utilization.
How can AI help reduce waste in manufacturing processes?
By analyzing data collected from sensors installed throughout a production line, AI algorithms can identify patterns that may indicate inefficiencies or sources of waste. This information can be used to adjust process parameters on-the-fly so that fewer resources are wasted during operations.
Can AI also improve the quality of manufactured products?
Yes, by using algorithms that detect defects or inconsistencies in parts produced by a machine, manufacturers can quickly make changes to improve product quality while reducing overall scrap rates.
Is it expensive for manufacturers to implement an AI-based waste reduction solution?
The cost of implementing an AI-based system will vary depending on the scale of your manufacturing operation and how many components you plan on using such technology with, but typically it requires a significant investment upfront – especially if sophisticated systems or custom hardware peripherals need integration with existing infrastructure.o however, companies might partner up with providers who specialize in these areas offering support & guidance which could mitigate costs over time while delivering measurable ROI & environmental benefits through reductions associated with pollution/labor overheads/energy consumption rates, etc.).