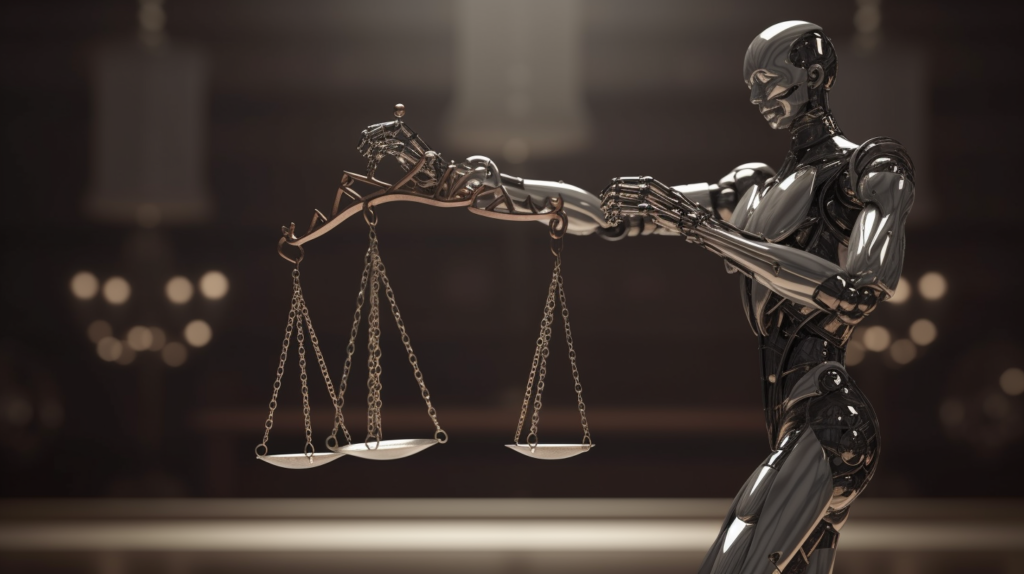
Artificial intelligence (AI) revolutionizes how businesses make decisions by offering real-time insights, predictive analytics, and data-driven solutions. AI can process vast amounts of data quickly and accurately, thus helping organizations identify trends, uncover hidden patterns, and anticipate future events. For instance, in finance or investment management industries, AI systems use advanced scoring algorithms and quantitative trading strategies to make more informed credit decisions with reduced human biases.
Furthermore, integrating AI into business intelligence (BI) applications allows organizations to access critical information faster and more efficiently than traditional methods.
These predictive models can offer personalized recommendations for optimal financial risk management while enhancing overall performance.
In summary, artificial intelligence enhances decision-making processes across various sectors by empowering businesses with accurate information from extensive data analysis facilitated through state-of-the-art technology.
Table of Contents
How Does Artificial Intelligence Affect Financial Decisions?
Artificial intelligence (AI) is revolutionizing the financial industry by enabling more accurate, data-driven decisions and transforming various aspects of finance. For example, AI can analyze vast amounts of financial data to spot trends and patterns that human analysts might miss, resulting in faster and more informed decision-making.
One prime example of AI’s impact on financial decisions is quantitative trading. In this area, hedge funds and investment firms use AI-powered tools like Kavout Corporation’s platform to process real-time market data alongside historical information for generating profitable trading strategies based on specific risk profiles.
Lastly, artificial intelligence contributes significantly towards fraud detection and prevention within the finance sector—safeguarding businesses from substantial losses due to fraudulent activities.
In summary, artificial intelligence plays a crucial role in shaping better financial decisions by offering comprehensive insights derived from complex datasets at rapid speeds while addressing potential biases prevalent among traditional methods involving human judgment alone—an invaluable asset for today’s evolving business landscape.
How Is AI Being Used In Finance?
Artificial Intelligence (AI) has been transforming the world of finance in several ways, bringing about significant improvements in various areas such as credit decisions, quantitative trading, and financial risk management.
For instance, AI-driven credit decision-making uses advanced algorithms to evaluate applicants’ creditworthiness by incorporating various information sources beyond traditional data sets.
This enables lenders to make more accurate assessments and reach underserved market segments.
In quantitative trading, AI empowers asset managers to quickly process vast amounts of data from multiple sources – far beyond human capabilities – resulting in algorithmic trading processes that automate trades for better efficiency.
Another key use case for AI within finance is its application in financial risk management. By automating complex analysis tasks required for assessing potential risks associated with investments or business decisions, companies can streamline their monitoring processes while reducing reliance on human judgment prone to biases and errors.
Overall, integrating artificial intelligence into modern-day finance has unlocked new possibilities for improved decision-making while optimizing operational efficiency across various aspects of this industry.
How Do Big Data And Artificial Intelligence Impact Financial Management Decisions?
Big data and artificial intelligence (AI) are revolutionizing the financial industry by providing new insights and tools for making smarter, more informed decisions. Financial organizations can now leverage vast information to identify previously hidden or inaccessible patterns, trends, and opportunities.
One prime example is AI-driven credit decision-making. Traditionally, credit decisions relied on basic metrics like income or credit scores to assess risk; however, incorporating artificial intelligence allows for more nuanced analysis through predictive analytics and advanced scoring algorithms.
Similarly, big data analytics and machine learning technologies have transformed quantitative trading strategies. Firms can now enhance investment management by mining colossal datasets covering everything from market trends to news articles.
For instance, companies like Kavout Corporation use sophisticated pattern recognition techniques combined with natural language processing tools to provide real-time signals for traders based on changing market conditions – paving the way toward a more agile approach to managing investments.
AI-Driven Decision Intelligence
AI-Driven Decision Intelligence involves using advanced algorithms and machine learning techniques to streamline access to data, simplify complex processes, and make accurate decisions in real time.
Streamlining Access To Data
AI-driven decision intelligence streamlines access to data in the financial industry, making it easier for businesses to make informed decisions. By using AI and business intelligence (BI) solutions like Tableau and Datapine, companies can quickly gather the information required and cut through the noise that would otherwise cloud human judgment.
BI-enabled businesses benefit from critical insights into data that were previously difficult to examine. With the help of AI-enabled BI applications, new data can be processed and trends identified through machine learning and predictive analytics.
Simplifying Complex Processes
Artificial intelligence has proven to be a game-changer in simplifying complex financial processes. By leveraging machine learning and predictive analytics, AI can process vast amounts of data faster than humans, identify patterns and trends, and generate insights that are not easily identifiable by traditional methods.
In addition to reporting capabilities, AI can help banks cut down on time-consuming manual tasks such as regulatory compliance checks. For example, banks must conform to several regulations across various jurisdictions globally; sifting through massive volumes of legal data is an inefficient use of resources.
However, risk management solutions such as Symphony AyasdiAI help automate this process and enable them to easily search for specific clauses within texts.
Use Cases Of AI In Financial Decision Making
AI is used in various areas of financial decision-making, including credit decisions, risk management, quantitative trading, personalized banking, fraud detection and cybersecurity, and blockchain banking.
Credit Decisions
AI is transforming the credit decision-making process in finance. With AI-powered underwriting platforms analyzing millions of data points, lenders can make more informed credit decisions with increased accuracy and speed.
Scienaptic AI, Zest AI, and Underwrite.ai are some examples of such platforms that use machine learning algorithms to assess borrowers’ risk profiles based on their income, spending habits, payment history, and other factors.
Thanks to advanced analytics technology available today, predicting future events has become more accurate than ever before.
Risk Management
AI has transformed risk management in the financial industry. Using predictive analytics, machine learning algorithms can identify potential risks and help companies mitigate them before they cause harm.
For instance, AI can detect fraudulent activity within a financial system by analyzing transactional patterns and spotting irregularities humans may overlook.
One example of AI being used for risk management is Morgan Stanley WealthDesk. The platform uses natural language processing (NLP) technology to analyze news articles, social media posts, filings, and other sources of information from around the world to generate investment recommendations for clients while minimizing their risk exposure.
Quantitative Trading
Using AI for quantitative trading has become increasingly popular in financial decision-making. By analyzing large amounts of complex data sets, AI-powered computers can make algorithmic trading processes much faster and more efficient than human-based ones.
For instance, Kensho Technologies uses machine learning to predict how various events affect stock markets and help traders make better investment decisions based on their analyses.
Likewise, AlphaSense uses natural language processing (NLP) algorithms to scan through SEC filings and other transcripts and identify relevant information that could influence a company’s stock price.
Personalized Banking
Personalized banking is a significant area where AI is transforming the finance industry. With the help of machine learning algorithms and predictive analytics, banks can offer personalized product recommendations in real time.
For instance, some mobile apps suggest personalized budgeting plans based on users’ spending habits.
Moreover, decision intelligence can provide real-time access to automated insights on individual customers’ asset and investment management portfolios. Banking institutions like Morgan Stanley use WealthDesk’s AI-driven workflows to automate repetitive tasks and deliver more sophisticated financial products to their clients.
Fraud Detection And Cybersecurity
AI is revolutionizing the financial industry with its ability to detect fraudulent activity and enhance cybersecurity. One example of this technology at work is Mastercard’s Decision Intelligence solution, which uses AI to analyze transactions in real time and provide a risk score that can help prevent fraud before it occurs.
Similarly, online finance companies employ automated threat detection and biometric authentication powered by machine learning algorithms to secure their customers’ accounts.
With AI constantly analyzing vast amounts of data, criminals have fewer opportunities to slip through the cracks undetected.
Blockchain Banking
Blockchain technology is revolutionizing the finance industry, and banking is no exception. As a result, banks can provide secure, transparent, and efficient services to their customers by using distributed ledgers to record transactions across multiple computers.
For example, Morgan Stanley’s WealthDesk platform uses machine learning algorithms to analyze client portfolios and suggest investments based on market trends and preferences.
Also, blockchain-based payment systems such as TQ Tezos allow faster cross-border transfers with lower fees while improving security through cryptographic authentication.
Advantages Of AI In Financial Decision Making
AI in financial decision-making offers improved accuracy, faster processing time, reduced costs, and enhanced customer experience.
Improved Accuracy
One of the biggest advantages of using AI in financial decision-making is improved accuracy. Unlike humans, AI-driven workflows can process vast amounts of data quickly and make unbiased decisions based on that data.
For instance, AI algorithms can analyze customer behavior to identify patterns and predict future purchases accurately. As a result, companies can tailor their marketing strategies accordingly, offering personalized recommendations to customers that increase sales while improving customer satisfaction.
Another example could be the use of AI in credit scoring systems, where machine learning models are used in assessing risk factors more accurately than traditional methods.
In conclusion, Improved Accuracy is one of the most notable benefits of using AI in financial decision-making today due to its ability to process vast amounts of data quickly without any biases, unlike human-led processes, which take time with an increased likelihood of mistakes or inaccurate information processing.
Faster Processing Time
AI’s ability to quickly process large amounts of data has revolutionized the financial industry, leading to faster processing times for various financial decisions.
For instance, AI can help banks analyze customer data instantly and accurately determine their creditworthiness, reducing the time it takes to approve loans from days to minutes.
Moreover, AI-powered decision analytics tools allow businesses to extract valuable information from complex datasets efficiently. This results in better-informed decisions being made at unprecedented speeds compared with traditional methods reliant upon human intervention.
Reduced Costs
Artificial intelligence has the potential to significantly reduce costs in financial decision-making. By automating processes and providing real-time insights, AI can help businesses make decisions more quickly and efficiently, resulting in lower operational costs.
For example, AI technology can perform credit scoring and underwriting faster than humans while detecting fraud with high accuracy rates. This reduces the time required for manual processing and saves money by avoiding costly mistakes caused by human error.
In addition, AI-powered quantitative trading can analyze large volumes of data faster than human traders while reducing investor transaction costs.
Overall, AI is transforming the finance industry towards a digital future where autonomous operations will enable better performance at a reduced cost.
Enhanced Customer Experience
Another advantage of using AI in financial decision-making is enhancing customer experience. By leveraging data analytics and artificial intelligence, businesses can better understand their customer’s needs and preferences, offering personalized banking services that cater to individual needs.
For instance, AI-driven chatbots can provide immediate responses to basic inquiries, delivering an optimal client service experience for retail banking users, with historical transactional information being available at their fingertips through computer algorithms correlated with behavioral profiles and trends analysis via big data analytics tools such as Datapine or Zoho Analytics.
The Future Of AI In Financial Decision Making
AI is expected to further integrate with other technologies, such as blockchain and quantum computing, leading to more advanced decision-making capabilities.
Ethical Considerations
The increasing use of AI in financial decision-making has raised important ethical considerations that must be considered. One major concern is the potential for AI to perpetuate or exacerbate existing inequalities.
This could occur if AI is trained using biased data or if its outputs result in differential treatment of certain groups. Therefore, it’s important to design AI with fairness and accountability principles to address this, ensuring transparency and oversight are present throughout its development and implementation.
Additionally, ongoing evaluation and adaptation should be incorporated to mitigate unintended consequences as they arise.
Integration With Other Technologies
AI is being integrated with other technologies to enhance financial security and transparency. One example is the integration of AI with blockchain technology, which creates a more secure network for financial transactions and minimizes fraud.
Additionally, cybersecurity measures are being reinforced through AI-driven algorithms that can identify potential threats before they cause damage.
According to important facts in the outline above, three of the largest accounting firms have pledged $9 billion toward AI and data analytics products and training, while by 2030, half of all large businesses are expected to have a full range of AI integrated into their processes.
Integrating AI with other technologies has significant implications for the future of finance, particularly regarding asset and investment management. For instance, integrating alternative data sources with predictive analytics software can provide real-time automated insights on individual portfolios and allow intelligent client outreach.
Conclusion
In conclusion, AI has transformed the financial industry by providing real-time insights and data-driven decision-making processes. From credit decisions to risk management, quantitative trading, and personalized banking experiences, artificial intelligence has streamlined complex processes while increasing accuracy and efficiency.
We can anticipate future events more accurately with advanced pattern recognition, predictive analytics, natural language processing, and deep learning models available at our fingertips.
As businesses continue to invest in AI-driven workflows and decision analytics solutions to empower autonomous operations that make better business decisions faster than humans ever could imagine doing alone or at scale – the benefits of a data culture emerge.
Integrating AI with blockchain technologies produces innovative solutions for payment security concerns while significantly improving asset management performance outcomes.
FAQ
How does AI assist in financial decision-making?
AI can analyze large amounts of financial data faster and more accurately than humans, identifying trends and insights that may not be apparent otherwise. This helps businesses make better-informed investments, risk management, and financial planning decisions.
What are some examples of AI tools used in financial decision-making?
Several AI tools are available for use in the finance industry, such as predictive analytics models, natural language processing (NLP), robotic process automation (RPA) systems, and chatbots.
Can AI replace human analysts when it comes to financial decision-making?
While AI can provide valuable insights based on data analysis, it cannot completely replace the judgment of an experienced human analyst with a deep understanding of market dynamics & ecosystems.
What steps should companies take before implementing an AI system for financial decision-making?
Before implementing any new technology solution like Artificial Intelligence within their organization, a company must conduct due diligence regarding its scalability, reliability metrics & working mechanism. Impact assessment studies should also be performed along with relevant safety protocols to mitigate potential risks arising from any issues that arise throughout usage over time. Additionally – staff training could help ensure a smooth transition during the introduction phase.